Conference Opening and Keynote Presentation
Sunday, October 13, 2024 |
9:00 - 10:00 |
Yasuda Auditorium |
Speaker
Dr. Miguel Hernan
Director
CAUSALab, Havard T.H. Chan School of Public Health
Causal estimands: Should we ask different causal questions in randomized trials and in the observational studies that emulate them?
Abstract
In comparative effectiveness and safety research, causal estimands are answers to questions that can be articulated by specifying a hypothetical randomized experiment: the target trial. Epidemiologists and others have precisely defined causal estimands when using observational data to emulate target trials. Recently, drug regulators have adopted a framework that defines causal estimands when using data from actual randomized trials. One might expect that both sets of estimands largely overlap because the results of observational studies are often compared with those from randomized trials, which implies that different study designs are used to try to answer similar causal questions. This talk considers causal estimands for comparative effectiveness and safety research, and why or why not they should be standardized across study designs.
Biography
Miguel Hernán uses health data and causal inference methods to learn what works. As Director of CAUSALab at Harvard, he and his collaborators repurpose real world data into evidence for the prevention and treatment of infectious diseases, cancer, cardiovascular disease, and mental illness. This work has contributed to shape health policy and research methodology worldwide.
Miguel is co-director of the Laboratory for Early Psychosis (LEAP) Center, principal investigator of the HIV-CAUSAL Collaboration, and co-director of the VA-CAUSAL Methods Core, an initiative of the U.S. Veterans Health Administration to integrate high-quality data and explicitly causal methodologies in a nationwide learning health system.
As the Kolokotrones Professor of Biostatistics and Epidemiology, he teaches at the Harvard T.H. Chan School of Public Health, where he has mentored dozens of trainees, and at the Harvard-MIT Division of Health Sciences and Technology. His free online course “Causal Diagrams” and book “Causal Inference: What If”, co-authored with James Robins, are widely used for the training of researchers.
Miguel has received several awards, including the Rousseeuw Prize for Statistics, the Rothman Epidemiology Prize, and a MERIT award from the U.S. National Institutes of Health. He is Fellow of the American Association for the Advancement of Science and the American Statistical Association, and Associate Editor of Annals of Internal Medicine. He is a former Special Government Employee of the U.S. Food and Drug Administration, Editor Emeritus of Epidemiology, and past Associate Editor of Biometrics, American Journal of Epidemiology, and Journal of the American Statistical Association.
Moderator
Hiraku Kumamaru
Associate Professor
The University of Tokyo
Soko Setoguchi
Rutgers University
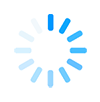