Concurrent Contributed Papers Session: Methods
Tracks
Track 1
Sunday, October 13, 2024 |
13:00 - 14:30 |
Yasuda Auditorium |
Speaker
Meng Zhang
Peking University
Research status of applying common data model in pharmacoepidemiology: a systematic review
13:00 - 13:15Abstract
Introduction
The transformation of medical data into the common data model (CDM) enables multi-center collaborative research, enhancing the ability to address questions regarding drug effectiveness and safety. However, the application of the CDM in pharmacoepidemiologic research remain unclear.
Aims
To systematically summarize global work on the use of CDM in pharmacoepidemiologic research.
Methods
Five English databases (PubMed, Web of Science, EMBASE, Scopus, Virtual Health Library) and four Chinese databases (CNKI, Wan-Fang Data, VIP, SinoMed) were electronically searched to collect relevant studies on applying CDM in pharmacoepidemiologic research from inception to January 2024. Two reviewers independently screened studies and extracted information on research details, CDM-related, pharmacoepidemiology-related information, and whether reporting guidelines were applied.
Results
This study included 309 studies, with 308 in English and 1 in Chinese. The median number of centers was 7 (IQR 4-8), with a median sample size of 267,182 (IQR 16,228-1,531,144). The top 3 CDMs used were VSD (52.8%), OMOP (24.3%), and Sentinel (6.1%). 79.9% of the studies utilized US data sources. Korea (18.4%), China (2.6%), and Japan (2.6%) contributed part of Asian data. The focus was on vaccines in 56.0% of the studies, drugs in 43.4%, and devices/surgeries in 0.6%. The most commonly studied vaccines were influenza vaccines (54/173) and COVID-19 vaccines (21/173). Primary drugs included antidiabetic drugs (21/134) and antibiotics (12/134). Research directions predominantly encompassed safety (77.3%), drug/vaccine utilization (17.2%), effectiveness (8.0%), and others. Safety events were primarily concentrated on the nervous systems diseases (58/237) and autoimmune diseases (49/237). Only 16 studies used reporting guidelines.
Discussion
Our study provides a comprehensive perspective on all CDMs, related drugs/vaccines, and treatment areas, identifying the feasibility of CDMs in pharmacoepidemiologic studies. However, the future direction of CDM applications still needs further expansion, with a focus on enhancing the standardization of research reports.
The transformation of medical data into the common data model (CDM) enables multi-center collaborative research, enhancing the ability to address questions regarding drug effectiveness and safety. However, the application of the CDM in pharmacoepidemiologic research remain unclear.
Aims
To systematically summarize global work on the use of CDM in pharmacoepidemiologic research.
Methods
Five English databases (PubMed, Web of Science, EMBASE, Scopus, Virtual Health Library) and four Chinese databases (CNKI, Wan-Fang Data, VIP, SinoMed) were electronically searched to collect relevant studies on applying CDM in pharmacoepidemiologic research from inception to January 2024. Two reviewers independently screened studies and extracted information on research details, CDM-related, pharmacoepidemiology-related information, and whether reporting guidelines were applied.
Results
This study included 309 studies, with 308 in English and 1 in Chinese. The median number of centers was 7 (IQR 4-8), with a median sample size of 267,182 (IQR 16,228-1,531,144). The top 3 CDMs used were VSD (52.8%), OMOP (24.3%), and Sentinel (6.1%). 79.9% of the studies utilized US data sources. Korea (18.4%), China (2.6%), and Japan (2.6%) contributed part of Asian data. The focus was on vaccines in 56.0% of the studies, drugs in 43.4%, and devices/surgeries in 0.6%. The most commonly studied vaccines were influenza vaccines (54/173) and COVID-19 vaccines (21/173). Primary drugs included antidiabetic drugs (21/134) and antibiotics (12/134). Research directions predominantly encompassed safety (77.3%), drug/vaccine utilization (17.2%), effectiveness (8.0%), and others. Safety events were primarily concentrated on the nervous systems diseases (58/237) and autoimmune diseases (49/237). Only 16 studies used reporting guidelines.
Discussion
Our study provides a comprehensive perspective on all CDMs, related drugs/vaccines, and treatment areas, identifying the feasibility of CDMs in pharmacoepidemiologic studies. However, the future direction of CDM applications still needs further expansion, with a focus on enhancing the standardization of research reports.
Biography
Meng Zhang is currently a doctoral candidate in the School of Public Health of Peking University. Her supervisor is Professor Feng Sun, and her main research directions are pharmacoepidemiology and real-world research. She has published 2 SCI papers as the first author/co-first author, and has presented oral presentations or poster presentations in 5 large academic conferences, such as ACPE 2023 and International Society for Pharmacoeconomics and Outcomes Research 2024.
Dr. Miki Ohta
Clinical Research Promotion Center
The utility of event-specific methods in causality assessment of drug-adverse reaction
13:15 - 13:30Abstract
Introduction:
Assessing the causal relationship between drugs and adverse events is a crucial step in pharmacovigilance. Suspected adverse drug reaction case reports from clinical practice to regulatory authorities often fail to assess causality due to a lack of information, particularly in the case of fatal cerebral haemorrhage. We have previously used a new algorithm (ACAD-FCH) to evaluate the causal relationship between drugs and fatal cerebral haemorrhage, and have examined the usefulness of this event-specific causal evaluation method.
Aims:
This study aimed to discuss the type of evaluation methods required to improve the quality of reports of suspected adverse drug reactions and the information needed for evaluation, based on an analysis of causality assessment of the fatal cerebral haemorrhage cases.
Methods:
The medical records of patients who died at the University of Tokyo Hospital in 2020 were reviewed, and cases with intracranial haemorrhage were selected. Two evaluators independently assessed these cases using three methods (ACAD-FCH, Naranjo algorithm, and WHO-UMC scale). The number of ‘Yes’, ‘No’, and ‘No information/Do not know’ responses were summed and compared. Inter-rater reliability was evaluated using agreement rates and kappa coefficients with 95% confidence intervals (CI).
Results:
Among 316 deaths, 24 cases with intracranial haemorrhage were evaluated. The proportion of ‘No information/Do not know’ responses was 35.6% (95% CI 31.4–40.6%) for ACAD-FCH and 66.9% (95% CI 62.5–71.1%) for the Naranjo algorithm. The agreement rates and kappa coefficients were 0.917 (0.798–1.00) and 0.867 (0.675–1.00) for ACAD-FCH, 0.708 (0.512–0.904) and 0.139 (−0.236 to 0.513) for the Naranjo algorithm, and 0.50 (0.284–0.716) and 0.326 (0.110–0.541) for the WHO-UMC scale.
Discussion:
For causality assessment between a drug and an adverse event, event-specific criteria and evaluation methods are preferable. Using event-specific methods may ensure appropriate reporting with the necessary information for causality assessment.
Assessing the causal relationship between drugs and adverse events is a crucial step in pharmacovigilance. Suspected adverse drug reaction case reports from clinical practice to regulatory authorities often fail to assess causality due to a lack of information, particularly in the case of fatal cerebral haemorrhage. We have previously used a new algorithm (ACAD-FCH) to evaluate the causal relationship between drugs and fatal cerebral haemorrhage, and have examined the usefulness of this event-specific causal evaluation method.
Aims:
This study aimed to discuss the type of evaluation methods required to improve the quality of reports of suspected adverse drug reactions and the information needed for evaluation, based on an analysis of causality assessment of the fatal cerebral haemorrhage cases.
Methods:
The medical records of patients who died at the University of Tokyo Hospital in 2020 were reviewed, and cases with intracranial haemorrhage were selected. Two evaluators independently assessed these cases using three methods (ACAD-FCH, Naranjo algorithm, and WHO-UMC scale). The number of ‘Yes’, ‘No’, and ‘No information/Do not know’ responses were summed and compared. Inter-rater reliability was evaluated using agreement rates and kappa coefficients with 95% confidence intervals (CI).
Results:
Among 316 deaths, 24 cases with intracranial haemorrhage were evaluated. The proportion of ‘No information/Do not know’ responses was 35.6% (95% CI 31.4–40.6%) for ACAD-FCH and 66.9% (95% CI 62.5–71.1%) for the Naranjo algorithm. The agreement rates and kappa coefficients were 0.917 (0.798–1.00) and 0.867 (0.675–1.00) for ACAD-FCH, 0.708 (0.512–0.904) and 0.139 (−0.236 to 0.513) for the Naranjo algorithm, and 0.50 (0.284–0.716) and 0.326 (0.110–0.541) for the WHO-UMC scale.
Discussion:
For causality assessment between a drug and an adverse event, event-specific criteria and evaluation methods are preferable. Using event-specific methods may ensure appropriate reporting with the necessary information for causality assessment.
Biography
EDUCATION
1993 - 1997 B.Soc.Sci and Family Studies, Ochanomizu University. Tokyo, Japan.
1997 - 2003 M.D., Kochi Medical School. Kochi, Japan.
2016 Ph.D., Saitama Medical School. Saitama, Japan
2019 - 2020 M.P.H., the University of Melbourne, Victoria, Australia
POSTGRADUATE TRAINING
Department of Neurosurgery, Tokyo University Hospital
Department of Neurosurgery, Teikyo University Hospital
Department of Neurosurgery, Teraoka Memorial Hospital
Department of Neurosurgery, Japanese Red Cross Medical Center
Department of Neurosurgery, Saitama Medical School
Phamaceuticals and Medical Devices Agency
QUALIFICATIONS
2003 - Japanese Medical License
2009 - Board Certification, The Japan Neurosurgical Society
2017 - Board Certification, Japan Board of Public Health and Social Medicine
Dr. Tanavij Pannoi
Lecturer
Drug Utilization Research Unit and Department of Pharmaceutical Care, School of Pharmacy, Walailak University, Thailand.
Performance of Matching with Oversample and Replacement Strategy for Imbalanced Real-world Dataset.
13:30 - 13:45Abstract
Introduction: Propensity score matching (PSM) is widely used in electronic medical record studies to create balanced treatment groups. However, the unbalanced comparators in clinical dataset might lead to a comparator bias. This imbalance often leads to models favoring the majority class, resulting in inadequate predictions for the minority class, specifically in false negative bias.
Aims: To evaluate the performance of PSM with oversampling and replacement strategy using electronic medical record databases.
Methods: A retrospective, new user-active comparator, cohort comparing Proton-pump inhibitors (PPIs) to Histamine-2 antagonists for Stroke events was applied to assess the performances with covariate balance with average standard mean difference (SMD), area under the receiver operating characteristic (ROC) curve, and bootstrapping bias with its bias standard error. The average effect of treatment on the treated (ATT) with hazard ratios were also compared among PSM models
Results: PSM with generalized boosted model (PSM-GBM) with replacement and oversample strategy produced estimates with better balanced covariates than PSM with 1:1 matching without replacement. The ROC was 0.81 for PSM-GBM. Moreover, the bootstrapping bias (0.018) is relatively small compared to the bootstrap standard error (0.189), indicating that the difference between the original coefficients and the mean coefficients from bootstrap resampling is not substantial. In PSM-GBM, the bias standard error being equal to the bootstrap standard error suggests that there might be variability in the bias estimate across bootstrap samples. Nevertheless, the ATT outcomes were not statistical difference among PSM models.
Discussion: Matching with replacement and oversample strategy performs well for this unbalanced dataset. Although, there may not be strong evidence of false negative bias based solely on this comparison, further investigation into the stability and robustness of the Cox proportional hazards model, potentially through additional validation methods, would be warranted to confirm the absence of bias.
Aims: To evaluate the performance of PSM with oversampling and replacement strategy using electronic medical record databases.
Methods: A retrospective, new user-active comparator, cohort comparing Proton-pump inhibitors (PPIs) to Histamine-2 antagonists for Stroke events was applied to assess the performances with covariate balance with average standard mean difference (SMD), area under the receiver operating characteristic (ROC) curve, and bootstrapping bias with its bias standard error. The average effect of treatment on the treated (ATT) with hazard ratios were also compared among PSM models
Results: PSM with generalized boosted model (PSM-GBM) with replacement and oversample strategy produced estimates with better balanced covariates than PSM with 1:1 matching without replacement. The ROC was 0.81 for PSM-GBM. Moreover, the bootstrapping bias (0.018) is relatively small compared to the bootstrap standard error (0.189), indicating that the difference between the original coefficients and the mean coefficients from bootstrap resampling is not substantial. In PSM-GBM, the bias standard error being equal to the bootstrap standard error suggests that there might be variability in the bias estimate across bootstrap samples. Nevertheless, the ATT outcomes were not statistical difference among PSM models.
Discussion: Matching with replacement and oversample strategy performs well for this unbalanced dataset. Although, there may not be strong evidence of false negative bias based solely on this comparison, further investigation into the stability and robustness of the Cox proportional hazards model, potentially through additional validation methods, would be warranted to confirm the absence of bias.
Biography
Tanavij Pannoi (潘孟堂)
EDUCATION
PhD 2023
International Health Program, National Yang Ming Chiao Tung University
M.P.H. 2012
College of Public Health Science, Chulalongkorn University, Thailand.
B.B.A. (Marketing) 2008
Sukhothai Thammathirat Open University, Thailand.
B.Pharm 2004
Faculty of Pharmaceutical Sciences, Prince of Songkla University, Thailand.
RESEARCH INTEREST
________________________________________
• Application of advanced methodology in assessing causal association between medication use and health outcomes, especially pharmacoepidemiology and pharmacoeconomic with longitudinal and/or administrative database.
• Drug Utilization Research
• Health care system and health policy research, particularly health financing.
Dr Md Jamal Uddin
Faculty
Shahjalal University of Science and Technology
Evaluation of Logistic Regression Model for Analyzing Pooled Observational Epidemiological Databases
13:45 - 14:00Abstract
Introduction:Pooling observational epidemiological databases increases sample size and improves accuracy of estimates. Two strategies exist for estimating parameters from pooled data: one-stage and two-stage. Although the traditional logistic regression model (LRM) is commonly used for single databases, its performance in pooled data is not well studied.
Aims:We aimed to assess the performance of LRM for analysing pooled epidemiological databases using simulated datasets.
Methods:We simulated 10 datasets with four scenarios: fixed intercept and slope with no differences between databases, fixed intercept and random slope indicating heterogeneity, random intercept and fixed slope, and random intercept and random slope. Sample sizes varied from 5000 to 12000, with a pooled size of about 60000. The outcome (Y) and exposure (X) were generated from the Bernoulli distribution, with Y prevalence of 3%, 20%, and 50%. Each scenario was replicated 1000 times. Two levels of variation (0.1 and 0.25) were introduced for random intercept and slope. Performance was measured by bias, RMSE, and coverage probability.
Results:When there is no heterogeneity between databases, one-stage models, particularly the weighted fixed effect LRM, perform best with the lowest RMSE (0.045) compared to random effect and two-stage models. With heterogeneity, fixed effect LRM show poor performance (coverage < 0.80), worsening with increased heterogeneity or outcome prevalence. Here, random effect LRM perform better. Unweighted random effect models have better RMSE but higher bias and coverage than weighted models. Two-stage unweighted random effect LRM show superior coverage (0.93) compared to one-stage (0.90). With 3% prevalence, two-stage models perform worst, but at 50% prevalence, the gap narrows, showing similar results.
Conclusion:The performance of LRM depends on research scenarios. Both one- and two-stage methods can yield similar results, but heterogeneity significantly influences performance. For heterogeneous databases, either one-stage or two-stage random effect unweighted LRM may be used, but the two-stage method is recommended.
Aims:We aimed to assess the performance of LRM for analysing pooled epidemiological databases using simulated datasets.
Methods:We simulated 10 datasets with four scenarios: fixed intercept and slope with no differences between databases, fixed intercept and random slope indicating heterogeneity, random intercept and fixed slope, and random intercept and random slope. Sample sizes varied from 5000 to 12000, with a pooled size of about 60000. The outcome (Y) and exposure (X) were generated from the Bernoulli distribution, with Y prevalence of 3%, 20%, and 50%. Each scenario was replicated 1000 times. Two levels of variation (0.1 and 0.25) were introduced for random intercept and slope. Performance was measured by bias, RMSE, and coverage probability.
Results:When there is no heterogeneity between databases, one-stage models, particularly the weighted fixed effect LRM, perform best with the lowest RMSE (0.045) compared to random effect and two-stage models. With heterogeneity, fixed effect LRM show poor performance (coverage < 0.80), worsening with increased heterogeneity or outcome prevalence. Here, random effect LRM perform better. Unweighted random effect models have better RMSE but higher bias and coverage than weighted models. Two-stage unweighted random effect LRM show superior coverage (0.93) compared to one-stage (0.90). With 3% prevalence, two-stage models perform worst, but at 50% prevalence, the gap narrows, showing similar results.
Conclusion:The performance of LRM depends on research scenarios. Both one- and two-stage methods can yield similar results, but heterogeneity significantly influences performance. For heterogeneous databases, either one-stage or two-stage random effect unweighted LRM may be used, but the two-stage method is recommended.
Biography
Dr. Md. Jamal Uddin is a faculty member in the Department of Statistics at Shahjalal University of Science & Technology (SUST), Sylhet, Bangladesh. He earned his PhD from Utrecht University, The Netherlands (2011-2014), focusing on statistical methods for unmeasured confounding in pharmacoepidemiology. He was a Postdoctoral fellow in Biostatistics at the University of Copenhagen, Denmark (2017-2019), and worked as a Statistician in Mental Health at University Hospital Copenhagen on the iPSYCH project. His research interests include developing statistical methods for public health and epidemiology, focusing on causal inference using observational studies, genetics, and complex survey data. He also researches non-communicable diseases, obesity indices, and childhood diseases. Dr. Uddin has published extensively, attended numerous conferences, and secured two research grants for projects in Bangladesh. Currently, he supervises eight MSc students and two PhD students at SUST.
Dr. Tengfei Lin
Shenzhen Institute of Advanced Technology, Chinese Academy of Sciences
Synchronization of time zero in pharmacoepidemiology studies using target trial emulation approach
14:00 - 14:15Abstract
Introduction: Many pharmacoepidemiology studies adopted target trial emulation approach in recent years, which was considered as a useful framework for avoiding potential sources of bias. However, there is evidence that key methodological details are missing from the report of such studies.
Aims: The objective for this meta-research study is to evaluate if the three key time points (i.e., meeting eligibility, initiating treatment, and starting follow-up) are aligned to avoid bias when emulating a target trial, and whether the authors described a solution to properly address potential bias when these three key time points are not aligned.
Methods: We performed a comprehensive literature search on PubMed, Embase, Web of Science till February 20, 2023 (We plan to include all eligible studies published from inception until May 2024, and we are now updating this research ). Studies were assessed for inclusion/exclusion by two independent reviewers based on a pre-defined eligibility criteria. Any disagreement was resolved by a third reviewer. Pharmacoepidemiology studies published in English language claiming to have used target trial emulation framework were included. Study characteristics and information regarding methodological elements were extracted and evaluated by two independent reviewers based on a predesigned form.
Results: Fifty-five pharmacoepidemiology studies were identified. In 15 (27.3%) studies, follow-up started at eligibility, but treatment strategy is assigned after follow-up, which may introduce immortal time bias and misclassification bias. Among them, only 7 (43.8%) studies described a solution to mitigate these biases.
Conclusions: Most studies did not explicitly define their target trial and failed to comply with the principles of target trial emulation framework. Methodological and reporting quality of pharmacoepidemiology studies using target trial emulation framework needs improvement. Our findings indicate that evidence-based guidelines for the conduct and reporting of such studies may be useful.
Aims: The objective for this meta-research study is to evaluate if the three key time points (i.e., meeting eligibility, initiating treatment, and starting follow-up) are aligned to avoid bias when emulating a target trial, and whether the authors described a solution to properly address potential bias when these three key time points are not aligned.
Methods: We performed a comprehensive literature search on PubMed, Embase, Web of Science till February 20, 2023 (We plan to include all eligible studies published from inception until May 2024, and we are now updating this research ). Studies were assessed for inclusion/exclusion by two independent reviewers based on a pre-defined eligibility criteria. Any disagreement was resolved by a third reviewer. Pharmacoepidemiology studies published in English language claiming to have used target trial emulation framework were included. Study characteristics and information regarding methodological elements were extracted and evaluated by two independent reviewers based on a predesigned form.
Results: Fifty-five pharmacoepidemiology studies were identified. In 15 (27.3%) studies, follow-up started at eligibility, but treatment strategy is assigned after follow-up, which may introduce immortal time bias and misclassification bias. Among them, only 7 (43.8%) studies described a solution to mitigate these biases.
Conclusions: Most studies did not explicitly define their target trial and failed to comply with the principles of target trial emulation framework. Methodological and reporting quality of pharmacoepidemiology studies using target trial emulation framework needs improvement. Our findings indicate that evidence-based guidelines for the conduct and reporting of such studies may be useful.
Biography
Dr. Tengfei Lin is an assistant researcher at Shenzhen Institute of Advanced Technology, Chinese Academy of Sciences. His main areas of interest are Pharmacoeconomics and Pharmacoepidemiology. Examples of his work include evaluation of the comparative effectiveness, safety and cost-effectiveness of antihypertensive drugs using real world evidence from both hypertension registries and large healthcare databases. His current research is funded by National Natural Science Foundation of China (NNSFC) and focuses on the use of target trial emulation framework in real-world studies of comparative effectiveness and safety.
Statistician Richuan Zheng
Statistician
Janssen Pharmaceutical K. K.
Baseline Adjustment to Compare Post-marketing Surveillance Study and Randomized Clinical Trial
14:15 - 14:30Abstract
Aim/Objective:
After the approval of a new drug in Japan, post-marketing surveillance (PMS) studies are conducted to primarily detect safety signals within a diverse patient population. In this study, our objective is to demonstrate how baseline adjustment influences the comparison results when comparing the safety and efficacy outcomes between PMS and randomized clinical trials (RCTs) via simulation study. Meanwhile, we propose a few causal inference approaches which can be considered to adjust the baseline imbalances for this kind of comparisons.
Methods:
To represent typical scenarios encountered in RCTs and PMS studies, we created a simulated dataset consisting of both baseline covariates and outcomes. We applied logistic regression models to generate efficacy and safety outcomes, considering the broader target population in the PMS setting. Causal inference methodologies, such as propensity score matching, g-formula, and ATT weighting, were employed to estimate adjusted results. Performance evaluation was based on bias and mean square error (MSE) measures.
Results:
Our simulation study revealed that g-formula and ATT weighting demonstrated better performance in mitigating bias. However, the naive method outperformed in terms of MSE. Notably, when the proportion of adverse events was extremely low, ATT weighting exhibited increased bias with the marginal effect of weighted outcome compared to other methods.
Conclusion:
Our simulation study quantitatively evaluated the impact of adjusting these baseline differences in comparing simulation data that mimic PMS and RCTs. Depending on the situation, our findings suggest that causal inference methods of g-formula or ATT weighting might be the alternative approach for this comparison. As PMS and RCTs are different sources of evidence, there would be other potential unmeasurable factors which cause the differences in outcomes. By adjusting the baseline imbalances, we would be able to focus on the remaining possible differences between PMS and RCTs.
After the approval of a new drug in Japan, post-marketing surveillance (PMS) studies are conducted to primarily detect safety signals within a diverse patient population. In this study, our objective is to demonstrate how baseline adjustment influences the comparison results when comparing the safety and efficacy outcomes between PMS and randomized clinical trials (RCTs) via simulation study. Meanwhile, we propose a few causal inference approaches which can be considered to adjust the baseline imbalances for this kind of comparisons.
Methods:
To represent typical scenarios encountered in RCTs and PMS studies, we created a simulated dataset consisting of both baseline covariates and outcomes. We applied logistic regression models to generate efficacy and safety outcomes, considering the broader target population in the PMS setting. Causal inference methodologies, such as propensity score matching, g-formula, and ATT weighting, were employed to estimate adjusted results. Performance evaluation was based on bias and mean square error (MSE) measures.
Results:
Our simulation study revealed that g-formula and ATT weighting demonstrated better performance in mitigating bias. However, the naive method outperformed in terms of MSE. Notably, when the proportion of adverse events was extremely low, ATT weighting exhibited increased bias with the marginal effect of weighted outcome compared to other methods.
Conclusion:
Our simulation study quantitatively evaluated the impact of adjusting these baseline differences in comparing simulation data that mimic PMS and RCTs. Depending on the situation, our findings suggest that causal inference methods of g-formula or ATT weighting might be the alternative approach for this comparison. As PMS and RCTs are different sources of evidence, there would be other potential unmeasurable factors which cause the differences in outcomes. By adjusting the baseline imbalances, we would be able to focus on the remaining possible differences between PMS and RCTs.
Biography
Richuan is a statistician served on both pharmacoepidemiology studies and clinical trials in the pharmaceutical industry. Richuan is happy to present her joint work with her colleagues to exchange the ideas/opinion with the academic and/or industry members on the topic proposed in the abstract.
Moderator
Kenneth Man
University College London
Takuhiro Yamaguchi
Tohoku University Graduate School of Medicine
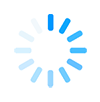