Concurrent Contributed Papers: Machine Learning / AI & Vaccinaton
Tracks
Track 1
Monday, October 14, 2024 |
16:00 - 17:30 |
Yasuda Auditorium |
Speaker
MSc Qi Sun
the University of Hong Kong
Machine learning for high-risk multimorbid antipsychotic user identification and cardiovascular event prediction
16:00 - 16:15Abstract
Introduction: Pre-existing multimorbidity is a known risk factor for major adverse cardiovascular events (MACE) among antipsychotic users. However, the association between multimorbidity and polypharmacy patterns and MACE remains to be clarified.
Aims: Identify specific multimorbidity patterns and antipsychotic use associated with increased MACE risks and develop and validate a time-to-event prediction model.
Methods: This retrospective cohort study utilized electronic health records from the Hong Kong CDARS database. Patients aged 18-65 years, with records of 2 or more chronic health conditions within three years prior to the initial date of antipsychotic intake, were enrolled. Baseline characteristics, including age, sex, chronic disease history, antipsychotic usage history, and previous one-year drug intake history, were collected. The dataset was randomly divided into training and validation subsets based on the initial year of antipsychotic prescription. A Conditional Inference Survival Tree (CISTree) was employed to classify MACE risk groups. Eight machine learning models were trained using 5-fold cross-validation for hyperparameter optimization and validated on the validation set.
Results: 27,466 patients were included. The CISTree model identified older patients (>44 years) with chronic kidney disease (CKD), cancer, hypertension, and using antianginal and antiplatelet drugs but not taking antidepressants as having the highest MACE incidence rate (173.065 per 1,000 person-years; 95% CI: [125.023, 230.990]). The Random Survival Forest (RSF) model outperformed the other seven models, identifying age, antidepressant usage, and chronic kidney disease (CKD) as the top three significant predictors. Furthermore, factors associated with a lower risk of MACE included younger age (<44 years), the use of antianginal, antibacterial, or antidepressant drugs, no usage of antiplatelet drugs or haloperidol, and the absence of CKD.
Discussion: We identified highly specific high-risk groups in multimorbidity people using antipsychotics; prediction based on the same features demonstrates excellent power and potential in aiding clinical decisions.
Aims: Identify specific multimorbidity patterns and antipsychotic use associated with increased MACE risks and develop and validate a time-to-event prediction model.
Methods: This retrospective cohort study utilized electronic health records from the Hong Kong CDARS database. Patients aged 18-65 years, with records of 2 or more chronic health conditions within three years prior to the initial date of antipsychotic intake, were enrolled. Baseline characteristics, including age, sex, chronic disease history, antipsychotic usage history, and previous one-year drug intake history, were collected. The dataset was randomly divided into training and validation subsets based on the initial year of antipsychotic prescription. A Conditional Inference Survival Tree (CISTree) was employed to classify MACE risk groups. Eight machine learning models were trained using 5-fold cross-validation for hyperparameter optimization and validated on the validation set.
Results: 27,466 patients were included. The CISTree model identified older patients (>44 years) with chronic kidney disease (CKD), cancer, hypertension, and using antianginal and antiplatelet drugs but not taking antidepressants as having the highest MACE incidence rate (173.065 per 1,000 person-years; 95% CI: [125.023, 230.990]). The Random Survival Forest (RSF) model outperformed the other seven models, identifying age, antidepressant usage, and chronic kidney disease (CKD) as the top three significant predictors. Furthermore, factors associated with a lower risk of MACE included younger age (<44 years), the use of antianginal, antibacterial, or antidepressant drugs, no usage of antiplatelet drugs or haloperidol, and the absence of CKD.
Discussion: We identified highly specific high-risk groups in multimorbidity people using antipsychotics; prediction based on the same features demonstrates excellent power and potential in aiding clinical decisions.
Biography
RA at the University of Hong Kong, master of Epidemiology (specializing in medical statistics) from Utrecht University, NL
Dr. Krishna Undela
Assistant Professor
Assistant Professor, NIPER Guwahati, INDIA
Artificial Intelligence Techniques for the Survival Prediction of Oropharyngeal Squamous Cell Carcinoma
16:15 - 16:30Abstract
Introduction: Oropharyngeal squamous cell carcinoma (OPSCC) is prevalent globally. Artificial intelligence techniques like deep learning and machine learning can be used for survival prediction with better accuracy than traditional models.
Aims: This study aimed to use machine learning and deep learning techniques to develop models for identifying and visualizing significant predictors of OPSCC survival rates.
Methods: A retrospective cohort study was conducted at a tertiary care cancer hospital in northeast India and retrieved a dataset of 1042 OPSCC patients admitted between 2018 and 2020. The dataset contained 12 predictor variables (independent variables) associated with the survival status (dependent variable) of the patients (alive or dead). Logistic regression, support vector machine (SVM), random forest (RF), and multilayer perceptron (MLP) were the prediction models used to determine the significant predictors of OPSCC survival rate. The key variables were ranked using random forest variable selection techniques. In addition to using the random forest technique, we also performed binary logistic regression to further evaluate the significance of these variables in predicting survivability. The study used various metrics to assess the classification performance of the models, including sensitivity, specificity, accuracy, precision, F1 score and Matthews’ correlation coefficient.
Results: The MLP model achieved the best performance across all evaluation metrics in this study. The MLP model was observed to have higher accuracy (83.25%; area under the curve (AUC)=0.83) and precision (0.83) than RF (accuracy=81.34%; AUC=0.80; precision=0.80), logistic regression (accuracy=77.99%; AUC=0.78; precision=0.75) and the lowest in SVM (accuracy=75.12%; AUC=0.75; precision=0.70). Variables such as age, treatment (surgery followed by radiotherapy), metastasis, T stage, and nodal status were identified as predictors of the OPSCC survival rate.
Conclusion: A deep learning technique incorporating the clinical variable can be an excellent model for predicting survival rates among OPSCC patients. The MLP model was observed to have better predictability than other models.
Aims: This study aimed to use machine learning and deep learning techniques to develop models for identifying and visualizing significant predictors of OPSCC survival rates.
Methods: A retrospective cohort study was conducted at a tertiary care cancer hospital in northeast India and retrieved a dataset of 1042 OPSCC patients admitted between 2018 and 2020. The dataset contained 12 predictor variables (independent variables) associated with the survival status (dependent variable) of the patients (alive or dead). Logistic regression, support vector machine (SVM), random forest (RF), and multilayer perceptron (MLP) were the prediction models used to determine the significant predictors of OPSCC survival rate. The key variables were ranked using random forest variable selection techniques. In addition to using the random forest technique, we also performed binary logistic regression to further evaluate the significance of these variables in predicting survivability. The study used various metrics to assess the classification performance of the models, including sensitivity, specificity, accuracy, precision, F1 score and Matthews’ correlation coefficient.
Results: The MLP model achieved the best performance across all evaluation metrics in this study. The MLP model was observed to have higher accuracy (83.25%; area under the curve (AUC)=0.83) and precision (0.83) than RF (accuracy=81.34%; AUC=0.80; precision=0.80), logistic regression (accuracy=77.99%; AUC=0.78; precision=0.75) and the lowest in SVM (accuracy=75.12%; AUC=0.75; precision=0.70). Variables such as age, treatment (surgery followed by radiotherapy), metastasis, T stage, and nodal status were identified as predictors of the OPSCC survival rate.
Conclusion: A deep learning technique incorporating the clinical variable can be an excellent model for predicting survival rates among OPSCC patients. The MLP model was observed to have better predictability than other models.
Biography
Krishna Undela has experience in conducting various Pharmacoepidemiological studies in hospital and community settings. He received the 2015 Endeavour Executive Fellowship from the Department of Education, Australian Government, to undertake three months of training on “Advanced Pharmacoepidemiology and Quality Use of Medicines” at the University of South Australia, Adelaide, Australia. He received travel grants from the International Society for Pharmacoepidemiology (ISPE) to attend ISPE conferences held in Hong Kong (in 2013), Taiwan (in 2014), Thailand (in 2015), and the USA (in 2021) as a delegate, and Australia (in 2017), China (in 2018), Japan (in 2019), Korea (in 2021), Taiwan (in 2022), and India (in 2023) as an Educational Session Speaker. He also received a travel grant from the International Society for Pharmacoeconomics and Outcomes Research (ISPOR) to attend the ISPOR European Congress held in Austria in 2016.
vismitha varghese
Student
NIPER Guwahati
Computational drug repurposing for gallbladder cancer integrated with pharmacovigilance approach
16:30 - 16:45Abstract
Introduction: Over 115,000 people die from gallbladder cancer (GBC) annually, a rare yet highly fatal disease accounting for 80-95% of biliary tract cancers. Its high recurrence rate, geographic disparities, limited treatments, and drug resistance underscore the urgent need for targeted therapies.
Aims. To identify potential targets and inverse pharmacovigilance signals associated with GBC and screen existing drugs for repurposing based on the identified targets.
Methods: A systematic search was conducted in Gene Expression Omnibus (GEO), and microarray expression profile datasets related to GBC were downloaded. Differentially expressed genes (DEGs) between normal and GBC were identified using GEO2R and Venn diagram tools, with those displaying a log (fold change) >1 and a p-value <0.05 considered significant. A protein-protein interaction network was built using the Search Tool for the Retrieval of Interacting Genes (STRING) and analyzed using Cytoscape. The prognostic significance of the target gene was evaluated using GEPIA, and genomic alterations were investigated through the cBioPortal database. Subsequently, inverse disproportionality analysis was performed using Open Vigil 2.1 on the FAERS database (to retrieve repurposable drugs). Furthermore, molecular docking was conducted in Biovia Discovery Studio by keeping dinaciclib (CDK1 inhibitor) as a reference to investigate the target’s interaction with the identified drugs.
Results: Cyclin-dependent Kinase 1 (CDK1), significantly overexpressed in GBC, was identified as a potential target. According to the GEPIA database, overexpression of CDK1 is associated with reduced survival of GBC patients. Among 54 drugs identified from inverse disproportionality analysis, levothyroxine and folic acid were prioritized due to their highest docking score of -77.83 Kcal/mol and -73.27 Kcal/mol, respectively, followed by atorvastatin and methotrexate. These findings hold promise for the future of GBC treatment.
Discussion: In the realm of drug repurposing, GBC is unexplored. Despite its aggressive nature, studies related to the molecular mechanism of the disease and targeted therapy are limited; our pioneering work, which takes a novel approach to drug repurposing, is the first to examine drug repurposing and identified levothyroxine, folic acid, atorvastatin, and methotrexate as promising candidates, highlighting the need for in vitro and in vivo studies.
Aims. To identify potential targets and inverse pharmacovigilance signals associated with GBC and screen existing drugs for repurposing based on the identified targets.
Methods: A systematic search was conducted in Gene Expression Omnibus (GEO), and microarray expression profile datasets related to GBC were downloaded. Differentially expressed genes (DEGs) between normal and GBC were identified using GEO2R and Venn diagram tools, with those displaying a log (fold change) >1 and a p-value <0.05 considered significant. A protein-protein interaction network was built using the Search Tool for the Retrieval of Interacting Genes (STRING) and analyzed using Cytoscape. The prognostic significance of the target gene was evaluated using GEPIA, and genomic alterations were investigated through the cBioPortal database. Subsequently, inverse disproportionality analysis was performed using Open Vigil 2.1 on the FAERS database (to retrieve repurposable drugs). Furthermore, molecular docking was conducted in Biovia Discovery Studio by keeping dinaciclib (CDK1 inhibitor) as a reference to investigate the target’s interaction with the identified drugs.
Results: Cyclin-dependent Kinase 1 (CDK1), significantly overexpressed in GBC, was identified as a potential target. According to the GEPIA database, overexpression of CDK1 is associated with reduced survival of GBC patients. Among 54 drugs identified from inverse disproportionality analysis, levothyroxine and folic acid were prioritized due to their highest docking score of -77.83 Kcal/mol and -73.27 Kcal/mol, respectively, followed by atorvastatin and methotrexate. These findings hold promise for the future of GBC treatment.
Discussion: In the realm of drug repurposing, GBC is unexplored. Despite its aggressive nature, studies related to the molecular mechanism of the disease and targeted therapy are limited; our pioneering work, which takes a novel approach to drug repurposing, is the first to examine drug repurposing and identified levothyroxine, folic acid, atorvastatin, and methotrexate as promising candidates, highlighting the need for in vitro and in vivo studies.
Biography
I am Vismitha Varghese, a dedicated Pharmacy Practice student at NIPER Guwahati. My academic journey has equipped me with comprehensive knowledge and hands-on experience in various aspects of pharmacy practice, including patient counseling, pharmacovigilance, and drug repurposing. I have a strong passion for cancer therapies, with a specific focus on developing innovative treatments for gallbladder cancer. My recent research involves computational drug repurposing integrated with a pharmacovigilance approach, aimed at identifying cost-effective and efficient treatments. Throughout my studies, I have actively engaged in research projects, manuscript writing, and have contributed to scientific journals. My commitment to advancing cancer therapies drives me to continuously seek new knowledge and contribute to the scientific community. I am eager to share my findings and insights at this conference, hoping to collaborate with fellow researchers and professionals in the field.
Dr. Grace Wangge
Associate Professor
Monash University, Indonesia
AI in Pharmacovigilance: Asia Pacific's Scientific and Public Discourse
16:45 - 17:00Abstract
Introduction: Artificial intelligence (AI) is transforming pharmacovigilance(PV) worldwide, but discussions are limited to Global North countries.
Aims: This study explores how the public and scientific communities in Asia Pacific countries discuss AI in PV, aiming to identify potential gaps and research focus.
Methods: We analysed online media conversations (2022-February 2024) and scientific literature from global and Asian databases to understand these discourse.
Results:
Our initial analysis of over 19,000 online conversations showed most discussions came from news sources, with limited (26 tweets) on social media platform X. These tweets focused on US FDA and South Korea collaboration on AI in medical products. Sentiment was neutral.
In the scientific literature, we found 17 relevant studies, mostly published in 2023 and originating from Japan (5), South Korea (4), and China (3). None were from South or Southeast Asian countries. The most frequently discussed AI techniques were machine learning (10/17) and natural language processing (NLP) (10/17). These studies primarily focused on using AI to detect adverse drug reactions (ADRs) (8/17), with less attention given to processing safety reports (2/17) or integrating prediction uncertainties (2/17). Challenges like data quality and management (6/17), model accuracy and adaptability (4/17), and integration with existing systems (3/17) were highlighted. Other potential applications of AI in pharmacovigilance, such as extracting drug-drug interactions, identifying high-risk patient groups, predicting side effects, and simulating clinical trials, were rarely or not at all explored. Ten studies expressed optimism about the positive impact of AI on drug safety and healthcare efficiency, while the remaining 7 acknowledged the potential but emphasized current limitations(neutral sentiment).
Conclusion: Discussions on potential of AI in PV, public and scientific, are still limited in the Asia Pacific region, particularly in South and Southeast Asia. This lack of representation suggests potential inequities in AI-driven drug safety solutions in the region.
Aims: This study explores how the public and scientific communities in Asia Pacific countries discuss AI in PV, aiming to identify potential gaps and research focus.
Methods: We analysed online media conversations (2022-February 2024) and scientific literature from global and Asian databases to understand these discourse.
Results:
Our initial analysis of over 19,000 online conversations showed most discussions came from news sources, with limited (26 tweets) on social media platform X. These tweets focused on US FDA and South Korea collaboration on AI in medical products. Sentiment was neutral.
In the scientific literature, we found 17 relevant studies, mostly published in 2023 and originating from Japan (5), South Korea (4), and China (3). None were from South or Southeast Asian countries. The most frequently discussed AI techniques were machine learning (10/17) and natural language processing (NLP) (10/17). These studies primarily focused on using AI to detect adverse drug reactions (ADRs) (8/17), with less attention given to processing safety reports (2/17) or integrating prediction uncertainties (2/17). Challenges like data quality and management (6/17), model accuracy and adaptability (4/17), and integration with existing systems (3/17) were highlighted. Other potential applications of AI in pharmacovigilance, such as extracting drug-drug interactions, identifying high-risk patient groups, predicting side effects, and simulating clinical trials, were rarely or not at all explored. Ten studies expressed optimism about the positive impact of AI on drug safety and healthcare efficiency, while the remaining 7 acknowledged the potential but emphasized current limitations(neutral sentiment).
Conclusion: Discussions on potential of AI in PV, public and scientific, are still limited in the Asia Pacific region, particularly in South and Southeast Asia. This lack of representation suggests potential inequities in AI-driven drug safety solutions in the region.
Biography
Dr. Grace Wangge is a medical doctor and epidemiologist dedicated to Public Health Policy and Drug Development. She joined Monash University Indonesia as an Associate Professor in the Master’s of Public Health program in 2022, following extensive roles at Universitas Indonesia and other institutions since 2003. Her research delves into public health, digital health governance, pharmacovigilance, and health science communication, with a focus on addressing challenges in low- and middle-income countries and during health emergencies such as the COVID-19 pandemic. Dr. Wangge has contributed to numerous peer-reviewed publications and policy briefs that discuss public health issues in Indonesia and Southeast Asia. She also serves as Vice President of the International Society of Pharmacovigilance's Indonesia Chapter since 2021 and has been actively engaged with the International Society of Pharmacoepidemiology since 2010.
Ms. Haerin Cho
Ewha womans university
Risk of Guillain-Barre syndrome after Influenza Vaccination in Korean Adults
17:00 - 17:15Abstract
Introduction:
Previous studies on the association between the influenza vaccine and Guillain-Barre syndrome (GBS) have shown varying results by study year and region.
Aims:
To evaluate the risk of GBS following influenza vaccination among Korean adults in the 2016-2017 to 2021-2022 seasons.
Methods:
We performed a self-controlled risk interval study using vaccination registry from the Korea Disease Control and Prevention Agency and the National Health Insurance Service claims data. This study included adults aged 18 and older who received a single dose of the influenza vaccine in at least one season from 2016 to 2022, with each season defined as the period from September to April of the following year. GBS event was defined as the first hospitalization for GBS within 84 days post-vaccination and was identified using both ICD-10 code (G61.0) and codes indicating treatment for GBS, a rare incurable disease. The exposure risk intervals were defined as 1–42 days post-vaccination with the control interval set at 43–84 days post-vaccination. Conditional Poisson regression model was used to estimate the adjusted incidence rate ratio (aIRR) with adjustments for calendar time by weekly interval.
Results:
The GBS cases identified within 84 days post-vaccination during the 2016-2017 to 2021-2022 seasons were 35, 27, 35, 58, 62, and 68, respectively. Corresponding aIRRs for the 2016-2017 season were 4.09 (95% confidence interval [CI]: 0.68–24.78); for the 2017-2018 season, 0.69 (95% CI: 0.12–4.06); for the 2018-2019 season, 0.41 (95% CI: 0.08–2.01); for the 2019-2020 season, 0.69 (95% CI: 0.19–2.48); for the 2020-2021 season, 0.75 (95% CI: 0.23–2.49); and for the 2021-2022 season, 1.70 (95% CI: 0.60–4.80).
Discussion/Conclusion:
There was no evidence of an increased risk of GBS after influenza vaccination during the 2016-2017 to 2021-2022 seasons. However, the recommended vaccine strain varies by season, continuous monitoring for GBS remains necessary.
Previous studies on the association between the influenza vaccine and Guillain-Barre syndrome (GBS) have shown varying results by study year and region.
Aims:
To evaluate the risk of GBS following influenza vaccination among Korean adults in the 2016-2017 to 2021-2022 seasons.
Methods:
We performed a self-controlled risk interval study using vaccination registry from the Korea Disease Control and Prevention Agency and the National Health Insurance Service claims data. This study included adults aged 18 and older who received a single dose of the influenza vaccine in at least one season from 2016 to 2022, with each season defined as the period from September to April of the following year. GBS event was defined as the first hospitalization for GBS within 84 days post-vaccination and was identified using both ICD-10 code (G61.0) and codes indicating treatment for GBS, a rare incurable disease. The exposure risk intervals were defined as 1–42 days post-vaccination with the control interval set at 43–84 days post-vaccination. Conditional Poisson regression model was used to estimate the adjusted incidence rate ratio (aIRR) with adjustments for calendar time by weekly interval.
Results:
The GBS cases identified within 84 days post-vaccination during the 2016-2017 to 2021-2022 seasons were 35, 27, 35, 58, 62, and 68, respectively. Corresponding aIRRs for the 2016-2017 season were 4.09 (95% confidence interval [CI]: 0.68–24.78); for the 2017-2018 season, 0.69 (95% CI: 0.12–4.06); for the 2018-2019 season, 0.41 (95% CI: 0.08–2.01); for the 2019-2020 season, 0.69 (95% CI: 0.19–2.48); for the 2020-2021 season, 0.75 (95% CI: 0.23–2.49); and for the 2021-2022 season, 1.70 (95% CI: 0.60–4.80).
Discussion/Conclusion:
There was no evidence of an increased risk of GBS after influenza vaccination during the 2016-2017 to 2021-2022 seasons. However, the recommended vaccine strain varies by season, continuous monitoring for GBS remains necessary.
Biography
Haerin Cho is a Ph.D student in pharmacoepidemiology at Ewha Womans University. She is interested in vaccines and epidemiologic methodologies utilizing real-world data, working full-time on related projects, and studying pharmacoepidemiology. She is also doing research with my colleagues to become a future contributor to advancing our country's regulatory science and risk management fields.
Ms. Chieh Yu Ho
School of Pharmacy, College of Pharmacy, Taipei Medical University
Trends and predictors of zoster vaccination coverage among U.S. cardiovascular disease population
17:15 - 17:30Abstract
Introduction:
Patients with cardiovascular disease have a higher risk of herpes zoster and subsequent cardiovascular events. The Centers for Disease Control and Prevention (CDC) recommends two zoster vaccines, Zostavax® and Shingrix®, for this population to prevent zoster and its complications. However, the zoster vaccination coverage and the predictors of vaccine uptake among patients with cardiovascular disease remain unknown.
Aims:
This study aimed to examine the trends of zoster vaccination and identify predictors of zoster vaccine uptake among adults with cardiovascular disease in the U.S.
Methods:
Data from the 2011 to 2022 National Health Interview Survey (NHIS) were used. NHIS is a nationally representative survey conducted annually to monitor the health of the U.S. population. The study population was adults aged ≥50 years with cardiovascular disease. Zoster vaccination was defined as respondents who self-reported having ever received the zoster vaccine. Weighted vaccination rates were estimated using NHIS survey design with clustering and stratification. Joinpoint regression was used to analyze changes in the direction and magnitude of trends. Multivariable logistic regression models were used to identify predictors associated with zoster vaccine uptake.
Results:
Zoster vaccination coverage significantly increased from 12.2% in 2011 to 40.5% in 2022 (p trend <0.01), with an average annual percentage change (AAPC) of 10.8%. One joinpoint at 2014 was identified. Predictors independently associated with zoster vaccine uptake included aged 70 years and over (p<0.05), females (p<0.05), college degree or higher education level (p<0.05), having health insurance (p<0.05), having a usual place of healthcare (p<0.05), and having a flu vaccination (p<0.05).
Discussion:
Our study indicated an increasing trend in zoster vaccination and identified several predictors of vaccine uptake among adults with cardiovascular disease in the U.S. These findings can assist healthcare providers in making informed decisions and implementing effective immunization strategies to promote zoster vaccination within this population.
Patients with cardiovascular disease have a higher risk of herpes zoster and subsequent cardiovascular events. The Centers for Disease Control and Prevention (CDC) recommends two zoster vaccines, Zostavax® and Shingrix®, for this population to prevent zoster and its complications. However, the zoster vaccination coverage and the predictors of vaccine uptake among patients with cardiovascular disease remain unknown.
Aims:
This study aimed to examine the trends of zoster vaccination and identify predictors of zoster vaccine uptake among adults with cardiovascular disease in the U.S.
Methods:
Data from the 2011 to 2022 National Health Interview Survey (NHIS) were used. NHIS is a nationally representative survey conducted annually to monitor the health of the U.S. population. The study population was adults aged ≥50 years with cardiovascular disease. Zoster vaccination was defined as respondents who self-reported having ever received the zoster vaccine. Weighted vaccination rates were estimated using NHIS survey design with clustering and stratification. Joinpoint regression was used to analyze changes in the direction and magnitude of trends. Multivariable logistic regression models were used to identify predictors associated with zoster vaccine uptake.
Results:
Zoster vaccination coverage significantly increased from 12.2% in 2011 to 40.5% in 2022 (p trend <0.01), with an average annual percentage change (AAPC) of 10.8%. One joinpoint at 2014 was identified. Predictors independently associated with zoster vaccine uptake included aged 70 years and over (p<0.05), females (p<0.05), college degree or higher education level (p<0.05), having health insurance (p<0.05), having a usual place of healthcare (p<0.05), and having a flu vaccination (p<0.05).
Discussion:
Our study indicated an increasing trend in zoster vaccination and identified several predictors of vaccine uptake among adults with cardiovascular disease in the U.S. These findings can assist healthcare providers in making informed decisions and implementing effective immunization strategies to promote zoster vaccination within this population.
Biography
My name is Chieh-Yu, Ho. I am a pharmacist serving at the pharmacy of Taipei Medical University Hospital, where I am responsible for cardiovascular-related services. Concurrently, I am pursuing a Master's degree in Clinical Pharmacy as an in-service student at Taipei Medical University, with a focus on the field of pharmacological epidemiology.
Moderator
Hiro Shintaro
Pfizer R&D Japan
Chi-Chuan Wang
National Taiwan University
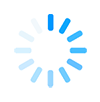