Tuesday Poster Presentations 2
Tuesday, September 23, 2025 |
12:00 PM - 1:30 PM |
Overview
Alternative Sampling Strategies, Analytical, Anti-Infective Drugs, Clinical Toxicology/ Drugs of Misuse, Immunosuppressive Drugs & Biomarkers, Pharmacogenetics, Pharmacometrics, TDM in Oncology and TDM of Biologics/Other
Speaker
Assist Prof Paweł K. Kunicki
Department Of Drug Chemistry, Pharmaceutical And Biomedical Analysis, Medical University Of Warsaw, Warsaw, Poland
Drug absorption in pulmonary arterial hypertension patients, one sampling time fits all?
Abstract
Background: The effectiveness of pharmacotherapy for pulmonary arterial hypertension (PAH) can be improved by monitoring adherence to treatment, including assessment of drug absorption after oral administration.
Aims: The aim was to determine the concentrations of sildenafil (SIL), bosentan (BOS), macitentan (MAC) and riociguat (RIO) in plasma collected from PAH patients before and after drug administration.
Methods: The study group was 85 PAH patients (27 M, 58 F), of whom 58 received SIL, 16 RIO, 28 BOS and 30 MAC. Blood samples were collected prior to (C0) and 3 h after drug administration (C3). HPLC-UV method was used for drug measuring in plasma. The analyzed drugs significantly differ in tmax value, therefore C3 usually meets the PK profile after (SIL and RIO) around (BOS) or before (MAC) reaching the maximum.
Results: Drug concentrations (with great variability) were determined in every sample, but in only 38 of 58 C0 samples for SIL. The median C0 was: 5.2, 83.5, 166.0, 183.2 ng/mL, while C3 was: 28.7, 155.6, 798.4, 296.4 ng/mL for SIL, RIO, BOS, MAC, respectively. Absorption expressed as (C3-C0) value was: 28.4±23.1 ng/mL (SIL), 45.5±53.5 ng/mL (RIO), 688.5±637.0 ng/mL (BOS) and 150.1±334.1 ng/mL (MAC). The swing (C3 instead of Cmax) showed: 801±829% (SIL), 93±94% (RIO), 1093±1765% (BOS) and 69±71% (MAC). Some negative values observed for (C3-C0) and swing indicate possible cases of non-adherence.
Conclusions: Paired C0 and C3 measurements is a useful tool to control PAH patient adherence. SIL monitoring cannot be successfully guided by C0.
Keywords: Sildenafil, Riociguat, Bosentan, Macitentan, Absorption
Aims: The aim was to determine the concentrations of sildenafil (SIL), bosentan (BOS), macitentan (MAC) and riociguat (RIO) in plasma collected from PAH patients before and after drug administration.
Methods: The study group was 85 PAH patients (27 M, 58 F), of whom 58 received SIL, 16 RIO, 28 BOS and 30 MAC. Blood samples were collected prior to (C0) and 3 h after drug administration (C3). HPLC-UV method was used for drug measuring in plasma. The analyzed drugs significantly differ in tmax value, therefore C3 usually meets the PK profile after (SIL and RIO) around (BOS) or before (MAC) reaching the maximum.
Results: Drug concentrations (with great variability) were determined in every sample, but in only 38 of 58 C0 samples for SIL. The median C0 was: 5.2, 83.5, 166.0, 183.2 ng/mL, while C3 was: 28.7, 155.6, 798.4, 296.4 ng/mL for SIL, RIO, BOS, MAC, respectively. Absorption expressed as (C3-C0) value was: 28.4±23.1 ng/mL (SIL), 45.5±53.5 ng/mL (RIO), 688.5±637.0 ng/mL (BOS) and 150.1±334.1 ng/mL (MAC). The swing (C3 instead of Cmax) showed: 801±829% (SIL), 93±94% (RIO), 1093±1765% (BOS) and 69±71% (MAC). Some negative values observed for (C3-C0) and swing indicate possible cases of non-adherence.
Conclusions: Paired C0 and C3 measurements is a useful tool to control PAH patient adherence. SIL monitoring cannot be successfully guided by C0.
Keywords: Sildenafil, Riociguat, Bosentan, Macitentan, Absorption
Biography
Paweł K. Kunicki, PhD,
He is (since 2016) - Assistant Professor at the Department of Drug Chemistry, Pharmaceutical and Biomedical Analysis, Faculty of Pharmacy, Medical University of Warsaw, Poland. He graduated as Master of Pharmacy (M.Pharm. 1990) and as Doctor in Pharmaceutical Sciences (Pharm.D., Ph.D. 2002). He was (1990–2019) - at the Institute of Cardiology in Warsaw, recently the Head of Clinical Pharmacology Unit, and acting Head of Department of Clinical Biochemistry.
He is a clinical pharmacist as well as a laboratory diagnostician, specialist in Public Health (2011) and in Laboratory Medical Toxicology (2021).
Consultant and expert on the field of drug analysis, pharmacokinetics and bioequivalence studies. He is an author of 53 original and review papers and of 202 oral or poster scientific presentations and lectures; h-index: 12. He is a member of IATDMCT (Immunosuppressive Drugs Committee), as well as Editorial Board member of Therapeutic Drug Monitoring.
Mrs Yuko Shimamoto
National Cerebral and Cardiovascular Center
Evaluation of apixaban dose reduction algorithm based on creatinine clearance levels
Abstract
Background
Dose reduction of apixaban (APX) is recommended for patients who meet at least two of the following three criteria: age ≥ 80 years, body weight (BW) ≤ 60 kg and serum creatinine (Scr) ≥ 1.5 mg/dL. The Scr-based criterion may overestimate renal function in elderly patients with low BW, potentially leading to overdosing.
Aims
This study aimed to compare the Scr criterion with the creatinine clearance (CLcr) criterion for APX dose reduction algorithms.
Methods
Patients who received 2.5 or 5.0 mg BID according to the Scr-based dose reduction algorithm were retrospectively enrolled. APX concentrations were measured by LC-MS/MS, and individual pharmacokinetic parameters were estimated using a Bayesian approach. A dosing simulation at the standard dose (5.0 mg BID) was performed, and the relationship between the simulated trough concentration and Scr levels was assessed. The CLcr-based dose reduction algorithm was simulated, and the number of patients with trough concentrations ≥ 230 ng/L was evaluated.
Results
Of the 84 patients, only 8 met the Scr criterion, and 40 received a reduced dose according to the Scr-based dose reduction algorithm. The simulated trough concentrations at the standard dose correlated poorly with Scr levels but strongly with CLcr, with 45 mL/min suggested as the cutoff value. The CLcr-based dose reduction algorithm decreased the number of patients with trough concentrations ≥ 230 ng/L from 12 to 10.
Conclusions
The CLcr criterion may be superior to the Scr criterion for the APX dose reduction algorithm, with limited impact in this population.
Keywords
Apixaban, Bayesian approach
Dose reduction of apixaban (APX) is recommended for patients who meet at least two of the following three criteria: age ≥ 80 years, body weight (BW) ≤ 60 kg and serum creatinine (Scr) ≥ 1.5 mg/dL. The Scr-based criterion may overestimate renal function in elderly patients with low BW, potentially leading to overdosing.
Aims
This study aimed to compare the Scr criterion with the creatinine clearance (CLcr) criterion for APX dose reduction algorithms.
Methods
Patients who received 2.5 or 5.0 mg BID according to the Scr-based dose reduction algorithm were retrospectively enrolled. APX concentrations were measured by LC-MS/MS, and individual pharmacokinetic parameters were estimated using a Bayesian approach. A dosing simulation at the standard dose (5.0 mg BID) was performed, and the relationship between the simulated trough concentration and Scr levels was assessed. The CLcr-based dose reduction algorithm was simulated, and the number of patients with trough concentrations ≥ 230 ng/L was evaluated.
Results
Of the 84 patients, only 8 met the Scr criterion, and 40 received a reduced dose according to the Scr-based dose reduction algorithm. The simulated trough concentrations at the standard dose correlated poorly with Scr levels but strongly with CLcr, with 45 mL/min suggested as the cutoff value. The CLcr-based dose reduction algorithm decreased the number of patients with trough concentrations ≥ 230 ng/L from 12 to 10.
Conclusions
The CLcr criterion may be superior to the Scr criterion for the APX dose reduction algorithm, with limited impact in this population.
Keywords
Apixaban, Bayesian approach
Biography
n/a
Dr Sarah Alghanem
College Of Pharmacy Of Kuwait University
Awareness, Attitudes, and Barriers to Clinical Utilization of Bayesian Dose Prediction Software
Abstract
Background: Bayesian dose prediction software is designed to aid clinicians in optimizing medication dosing in clinical settings.
Aims: This study evaluated clinicians' awareness and use of Bayesian dose prediction software, their attitudes toward its application, and the barriers to its clinical implementation, addressing the limited literature on its adoption in practice.
Methods: A cross-sectional, multi-organizational study was conducted using a validated electronic questionnaire distributed to 360 members across 8 organizations. Descriptive analyses were performed using SPSS version 28.
Results: The response rate was 65% (n=234) with most respondents from North America (67.4%). Forty-four participants who did not provide direct patient care were excluded, resulting in 190 eligible respondents. Among these, 82.6% (n=157) were aware of Bayesian dose prediction software, but only 65% (n=102) used it in their practice. The most commonly used software were InsightRx (40.2%), DoseMe (22.5%), and MWPharm (18.6%), primarily for dosing vancomycin (94.1%), aminoglycosides (50%), and tacrolimus (10.8%). The overall median (IQR) attitude score toward the software was 4.0 (2.0), indicating a positive attitude. The top three perceived barriers to clinical implementation among those aware of the software were the prohibitive cost of software licensing (65%), lack of healthy facility administrative support for the use and maintenance of the software (50.3%), and lack of awareness of how to use software into clinical practice (49.7%).
Conclusions: Findings indicate that while Bayesian dose prediction software is being utilized, its adoption remains limited to a few medications. The results highlight the need for targeted interventions to overcome the perceived barriers.
Aims: This study evaluated clinicians' awareness and use of Bayesian dose prediction software, their attitudes toward its application, and the barriers to its clinical implementation, addressing the limited literature on its adoption in practice.
Methods: A cross-sectional, multi-organizational study was conducted using a validated electronic questionnaire distributed to 360 members across 8 organizations. Descriptive analyses were performed using SPSS version 28.
Results: The response rate was 65% (n=234) with most respondents from North America (67.4%). Forty-four participants who did not provide direct patient care were excluded, resulting in 190 eligible respondents. Among these, 82.6% (n=157) were aware of Bayesian dose prediction software, but only 65% (n=102) used it in their practice. The most commonly used software were InsightRx (40.2%), DoseMe (22.5%), and MWPharm (18.6%), primarily for dosing vancomycin (94.1%), aminoglycosides (50%), and tacrolimus (10.8%). The overall median (IQR) attitude score toward the software was 4.0 (2.0), indicating a positive attitude. The top three perceived barriers to clinical implementation among those aware of the software were the prohibitive cost of software licensing (65%), lack of healthy facility administrative support for the use and maintenance of the software (50.3%), and lack of awareness of how to use software into clinical practice (49.7%).
Conclusions: Findings indicate that while Bayesian dose prediction software is being utilized, its adoption remains limited to a few medications. The results highlight the need for targeted interventions to overcome the perceived barriers.
Biography
Dr.Sarah Alghanem, is currently an Assistant Professor of Pharmacy Practice College of Pharmacy, Kuwait University. Her research focuses on clinical pharmacometrics (PKPD modelling and simulations) and outcome studies to evaluate and optimize the use of antimicrobial and immunosuppressant therapy. In addition, Dr.Alghanem is studying the development, implementation, and evaluation of advanced pharmacy services within the country. She is an active member at reginal and international organizations. She had significant contribution in shaping and transforming pharmacy profession in Kuwait by working closely with Kuwait Ministry of Health to expand pharmacy scope of practice and develop clinical pharmacy services policies and procedures to align with international standards.
Mrs Nana Xu
The First Affiliated Hospital Of Zhejiang University School Of Medicine
Understanding inter-individual variability of lurasidone pharmacokinetics: a population model in Chinese patients
Abstract
Background: Lurasidone is an effective treatment for bipolar disorder (BD), but its pharmacokinetics exhibit significant variability, leading to inconsistent therapeutic efficacy. Understanding the sources of this variability is crucial for optimizing treatment.
Aims: This study aimed to develop a population pharmacokinetic (PPK) model to investigate the impact of physiological and genetic factors on inter-individual variability of lurasidone.
Methods: BD patients who were dispensed lurasidone and underwent therapeutic drug monitoring were included. Clinical data, including demographics, genetic information (e.g., CYP3A4*1G, HTR1A), drug concentrations and medication information, were collected. A PPK model was developed using NONMEM software.
Results: A total of 241 plasma concentrations from 133 patients were adopted for PPK model development. A one-compartment model with first-order kinetics best described lurasidone’s pharmacokinetics. CYP3A4*1G genotype and height significantly influenced apparent clearance (CL/F) (P < 0.01), reducing variability from 40.5% to 36.7%. CL/F in CYP3A4*1G CC carriers (329 L/h) was 14.3% lower than that in TC carriers (384 L/h) and 25.4% lower than in TT carriers (441 L/h). However, HTR1A genotype was not notably associated with lurasidone clearance in our study.
Conclusions: Lurasidone PPK model in BD patients was established for the first time, hightlighting the effect of CYP3A4*1G genotype on pharmacokinetic variablility of lurasidone. This finding emphasizes the need for individualized dosing of lurasidone to optimize efficacy in BD treatment.
Keywords:lurasidone, cytochrome P450 3A4, population pharmacokinetics
Aims: This study aimed to develop a population pharmacokinetic (PPK) model to investigate the impact of physiological and genetic factors on inter-individual variability of lurasidone.
Methods: BD patients who were dispensed lurasidone and underwent therapeutic drug monitoring were included. Clinical data, including demographics, genetic information (e.g., CYP3A4*1G, HTR1A), drug concentrations and medication information, were collected. A PPK model was developed using NONMEM software.
Results: A total of 241 plasma concentrations from 133 patients were adopted for PPK model development. A one-compartment model with first-order kinetics best described lurasidone’s pharmacokinetics. CYP3A4*1G genotype and height significantly influenced apparent clearance (CL/F) (P < 0.01), reducing variability from 40.5% to 36.7%. CL/F in CYP3A4*1G CC carriers (329 L/h) was 14.3% lower than that in TC carriers (384 L/h) and 25.4% lower than in TT carriers (441 L/h). However, HTR1A genotype was not notably associated with lurasidone clearance in our study.
Conclusions: Lurasidone PPK model in BD patients was established for the first time, hightlighting the effect of CYP3A4*1G genotype on pharmacokinetic variablility of lurasidone. This finding emphasizes the need for individualized dosing of lurasidone to optimize efficacy in BD treatment.
Keywords:lurasidone, cytochrome P450 3A4, population pharmacokinetics
Biography
The author graduated with a Master's degree in Clinical Pharmacy from the Xiangya School of Pharmaceutical Sciences, Central South University in 2018. Since graduation, she has been working in the Department of Clinical Pharmacy at the First Affiliated Hospital of Zhejiang University School of Medicine, specializing in therapeutic drug monitoring (TDM) and personalized medication guidance. In 2023, she pursued advanced studies in population pharmacokinetic (PPK) modeling theory and practical implementation at Shanghai Jiao Tong University. Proficient in development and application of a LC-MS/MS Method for blood concentration detection, the author possesses extensive experience in establishing PPK models using drug concentration data to guide individualized dose adjustments. She has published 14 related SCI-indexed papers as first author or co-author.
Mr Hirohito Muroi
Kobe City Medical Center General Hospital
Comparison of 1-point and 2-point sampling for vancomycin Bayesian AUC estimation
Abstract
Background:
Vancomycin (VCM) therapeutic drug monitoring (TDM) has undergone a paradigm shift from trough-guided to AUC-guided TDM. Due to the clinical complexity of AUC measurement, Bayesian AUC estimation based on peak and trough sampling has been recommended in clinical practice. However, the actual impact of additional peak sampling on AUC estimation, compared to conventional trough sampling, remains unclear.
Aims:
This study aimed to compare Bayesian AUC estimations based on trough levels with those based on both peak and trough levels in clinical practice.
Methods:
Patients who had both peak and trough levels measured as part of routine TDM practices were retrospectively enrolled. Bayesian AUC estimations using both peak and trough levels (AUCpt) and trough levels only (AUCt) were performed with a published population pharmacokinetic model. The correlation between AUCt and AUCpt was calculated, and the AUCt ratio to AUCpt was assessed in relation to patient characteristics, dosing regimen, and the TDM timing.
Results:
A total of 208 patients were included. A strong correlation was observed between AUCpt and AUCt, and AUCt ratios ranged from 0.8 to 1.2. The AUCt ratio tended to increase with early TDM timing (<4 days), whereas no clear trend was observed in relation to patient characteristics or dosing regimen.
Conclusions:
Bayesian AUC estimations based on trough levels demonstrated comparable performance to those based on both peak and trough levels. The clinical benefits of additional peak sampling may be limited, particularly under steady-state conditions.
Keywords:
vancomycin, Bayesian estimation, TDM
Vancomycin (VCM) therapeutic drug monitoring (TDM) has undergone a paradigm shift from trough-guided to AUC-guided TDM. Due to the clinical complexity of AUC measurement, Bayesian AUC estimation based on peak and trough sampling has been recommended in clinical practice. However, the actual impact of additional peak sampling on AUC estimation, compared to conventional trough sampling, remains unclear.
Aims:
This study aimed to compare Bayesian AUC estimations based on trough levels with those based on both peak and trough levels in clinical practice.
Methods:
Patients who had both peak and trough levels measured as part of routine TDM practices were retrospectively enrolled. Bayesian AUC estimations using both peak and trough levels (AUCpt) and trough levels only (AUCt) were performed with a published population pharmacokinetic model. The correlation between AUCt and AUCpt was calculated, and the AUCt ratio to AUCpt was assessed in relation to patient characteristics, dosing regimen, and the TDM timing.
Results:
A total of 208 patients were included. A strong correlation was observed between AUCpt and AUCt, and AUCt ratios ranged from 0.8 to 1.2. The AUCt ratio tended to increase with early TDM timing (<4 days), whereas no clear trend was observed in relation to patient characteristics or dosing regimen.
Conclusions:
Bayesian AUC estimations based on trough levels demonstrated comparable performance to those based on both peak and trough levels. The clinical benefits of additional peak sampling may be limited, particularly under steady-state conditions.
Keywords:
vancomycin, Bayesian estimation, TDM
Biography
EDUCATION
• 2023-Present: Ph.D. in Pharmacy, Kobe Gakuin University, Hyogo
• 2015-2021: Bachelor of Pharmacy, Kobe Gakuin University, Hyogo
WORK EXPERIENCE
• April 2022-Present: Clinical Pharmacist, Kobe City Medical Center General Hospital
• April 2021-May 2022: Pharmacy Residency, Kobe City Medical Center General Hospital
Mr Masayoshi Kawata
Kyoto University Hospital
Propofol brain concentration during intraoperative awakening in awake craniotomy
Abstract
Background: During awake craniotomy, patients are awakened from anesthesia intraoperatively to allow for brain mapping and monitoring of brain functions. Therefore, individualized regulation of effect-site propofol concentration is crucial. However, there is significant inter-individual variability in the time requiring for intraoperative awakening.
Aims: This study aimed to establish a new pharmacokinetic model to describe plasma and brain propofol concentrations and to analyze the relationship between predicted concentrations and delayed intraoperative awakening.
Methods: Propofol concentrations in plasma and homogenized brain at 0, 5, 15, 25, and 75 min after cessation of administration to induce intraoperative awakening were quantified using HPLC with a fluorescence detector. Population pharmacokinetic modeling was conducted using NONMEM, based on the concentrations. Bispectral index (BIS), reflecting the depth of sedation, was extracted from surgical records. Delayed awakening was defined based on BIS values. Wilcoxon test was used to compare the predicted concentrations in patients with or without delayed awakening.
Results: A three-compartment model with effect-compartment and brain/plasma partition coefficient effectively described the concentrations. Delayed awakening was occured in 6 of 57 patients. The median predicted brain concentration at BIS>60 in the delayed-awakening patients was 1.03 µg/mL (range 0.16–4.41), which was significantly lower than that in the non-delayed patients (2.47 µg/mL, 0.18–7.79, P<0.05).
Conclusions: Given the concentrations at the time of awakening was sufficiently low in patients with delayed awakening, it is suggested that the delay is more likely due to pharmacodynamic factors rather than delayed drug elimination from pharmacokinetic reasons.
Key Words: Propofol, Brain concentration, Population pharmacokinetic model
Aims: This study aimed to establish a new pharmacokinetic model to describe plasma and brain propofol concentrations and to analyze the relationship between predicted concentrations and delayed intraoperative awakening.
Methods: Propofol concentrations in plasma and homogenized brain at 0, 5, 15, 25, and 75 min after cessation of administration to induce intraoperative awakening were quantified using HPLC with a fluorescence detector. Population pharmacokinetic modeling was conducted using NONMEM, based on the concentrations. Bispectral index (BIS), reflecting the depth of sedation, was extracted from surgical records. Delayed awakening was defined based on BIS values. Wilcoxon test was used to compare the predicted concentrations in patients with or without delayed awakening.
Results: A three-compartment model with effect-compartment and brain/plasma partition coefficient effectively described the concentrations. Delayed awakening was occured in 6 of 57 patients. The median predicted brain concentration at BIS>60 in the delayed-awakening patients was 1.03 µg/mL (range 0.16–4.41), which was significantly lower than that in the non-delayed patients (2.47 µg/mL, 0.18–7.79, P<0.05).
Conclusions: Given the concentrations at the time of awakening was sufficiently low in patients with delayed awakening, it is suggested that the delay is more likely due to pharmacodynamic factors rather than delayed drug elimination from pharmacokinetic reasons.
Key Words: Propofol, Brain concentration, Population pharmacokinetic model
Biography
Kyoto University Hospital, Kyoto (April 2012 - present)
Education: Bachelor of Pharmacy, Kyoto Pharmaceutical University, Kyoto (2012)
Dr Nan Hu
The Third Affiliated Hospital Of Soochow University
Population pharmacokinetics of tacrolimus in renal transplant recipients combined with posaconazole
Abstract
Background: Posaconazole can prevent and treat invasive fungal infections, but the combination will increase the blood concentration of tacrolimus, requiring dose adjustments to avoid side effects.
Aims: This study aimed to establish a PopPK model of tacrolimus in renal transplant patients combined with posaconazole.
Methods: We conducted a retrospective study of hospitalized patients who received posaconazole treatment or prevention of invasive fungal infection after renal transplantation in our hospital from January 2018 to December 2024, and performed pharmacokinetic analysis and Monte Carlo simulation.
Results: A total of 24 patients were included, and a one-compartment model of first-order absorption and elimination was established using the collected 202 blood samples to describe the pharmacokinetic characteristics of tacrolimus. The final model population typical values of tacrolimus apparent distribution volume (V/F) and apparent oral clearance rate (CL/F) were 957 L and 29.1 L/h, respectively. The key covariates in the final model included posaconazole combination time, serum creatinine, patient age, and CYP3A5 genotype. Monte Carlo simulations suggested initial tacrolimus doses of 9.5 mg for CYP3A5*1 carriers (*1/*1, *1/*3) and 8 mg for *3/*3 homozygotes before posaconazole initiation. During coadministration, doses required immediate reductions (21% for *1 carriers vs 31.25% for *3/*3), followed by daily 1 mg decreases for 2-4 days to maintain therapeutic levels (8-15 ng/mL).
Conclusions: A PopPK model of tacrolimus in renal transplant patients with posaconazole was developed and model-devised dosage regimens with tacrolimus concentrations provide a practical strategy for achieving the therapeutic range.
Keywords: tacrolimus; posaconazole; renal transplantation; PopPK; Monte Carlo simulations
Aims: This study aimed to establish a PopPK model of tacrolimus in renal transplant patients combined with posaconazole.
Methods: We conducted a retrospective study of hospitalized patients who received posaconazole treatment or prevention of invasive fungal infection after renal transplantation in our hospital from January 2018 to December 2024, and performed pharmacokinetic analysis and Monte Carlo simulation.
Results: A total of 24 patients were included, and a one-compartment model of first-order absorption and elimination was established using the collected 202 blood samples to describe the pharmacokinetic characteristics of tacrolimus. The final model population typical values of tacrolimus apparent distribution volume (V/F) and apparent oral clearance rate (CL/F) were 957 L and 29.1 L/h, respectively. The key covariates in the final model included posaconazole combination time, serum creatinine, patient age, and CYP3A5 genotype. Monte Carlo simulations suggested initial tacrolimus doses of 9.5 mg for CYP3A5*1 carriers (*1/*1, *1/*3) and 8 mg for *3/*3 homozygotes before posaconazole initiation. During coadministration, doses required immediate reductions (21% for *1 carriers vs 31.25% for *3/*3), followed by daily 1 mg decreases for 2-4 days to maintain therapeutic levels (8-15 ng/mL).
Conclusions: A PopPK model of tacrolimus in renal transplant patients with posaconazole was developed and model-devised dosage regimens with tacrolimus concentrations provide a practical strategy for achieving the therapeutic range.
Keywords: tacrolimus; posaconazole; renal transplantation; PopPK; Monte Carlo simulations
Biography
n/a
Mr Frank Sánchez Restrepo
Bioinstrumentation And Clinical Engineering Research Group- GIBIC, University of Antioquia, Medellin - Colombia
Reinforcement Learning for Generalized Dose Optimization
Abstract
Background: PopPK models are drug and population specific and combining them for model informed personalized dosing (MIPD) has thus far been limited to averaging dose recommendations from different models made for one drug in similar populations.
Aims: Use reinforcement learning (RL) to combine diverse popPK models for optimized dosing
Methods: We developed non-parametric population models for theophylline in adults and voriconazole in children and adult and from these models simulated 50 patients and concentration-time profiles for each drug after standard dosing, splitting the data into training (60%) and validation (40%) subsets. With one simulated concentration and set of covariates (drug, weight, age for voriconazole, drug for theophylline), we trained a deep RL agent to predict the optimal loading and maintenance doses to achieve specified concentration targets within a therapeutic window.
Results: In the validation data, the agent found loading and maintenance doses that achieved therapeutic concentrations in 90.5% of episodes overall. Specifically, therapeutic concentrations were achieved in 84% of episodes for voriconazole and 98% for theophylline.
Conclusions: We present a completely novel approach to MIPD that learns across drugs and populations, with subsequent creation of a single, generalized model where the drug itself becomes a covariate. Our results suggest that the method is feasible from typical popPK models and can be extended to more drugs and populations.
Keywords: Dose optimization, patient individualization, reinforcement learning.
Aims: Use reinforcement learning (RL) to combine diverse popPK models for optimized dosing
Methods: We developed non-parametric population models for theophylline in adults and voriconazole in children and adult and from these models simulated 50 patients and concentration-time profiles for each drug after standard dosing, splitting the data into training (60%) and validation (40%) subsets. With one simulated concentration and set of covariates (drug, weight, age for voriconazole, drug for theophylline), we trained a deep RL agent to predict the optimal loading and maintenance doses to achieve specified concentration targets within a therapeutic window.
Results: In the validation data, the agent found loading and maintenance doses that achieved therapeutic concentrations in 90.5% of episodes overall. Specifically, therapeutic concentrations were achieved in 84% of episodes for voriconazole and 98% for theophylline.
Conclusions: We present a completely novel approach to MIPD that learns across drugs and populations, with subsequent creation of a single, generalized model where the drug itself becomes a covariate. Our results suggest that the method is feasible from typical popPK models and can be extended to more drugs and populations.
Keywords: Dose optimization, patient individualization, reinforcement learning.
Biography
n/a
Dr Jordan Brooks
InsightRX
Lessons in Real-Time Communication of Potentially Erroneous Data in MIPD Software
Abstract
Background: Real-time communication of potential data errors in Model-Informed Precision Dosing (MIPD) software is crucial for clinical decision making, but it presents challenges. Flagging questionable data against a popPK model's residual error terms introduces biases, as some models have larger magnitude ("lenient") and others smaller magnitude ("stringent"). This study compares data flagging methods used in the InsightRX Nova platform.
Aim: Evaluate the impact of using drug-module-wide error magnitude versus model-specific error magnitude on data flagging and model selection bias.
Methods: We explored mechanisms for flagging questionable data based on residuals (IWRES), comparing model-specific residual error with a constant residual error magnitude across all popPK models for a given drug. This was conducted in both adult vancomycin (N = 55,646) and pediatric busulfan (N = 650) patients from the InsightRX Nova platform where data are flagged as “good”, “intermediate”, or “poor” fit in relation to their calculated residual.
Results: Using model-specific residual error magnitude for flagging questionable observations may lead to user selection bias towards more lenient models, even if those models are less accurate for a patient. Switching to module-wide residual error magnitude reduced bias and provided more consistent flagging across stringent and lenient models. In adult vancomycin data, "poor" flags decreased by 16.9%, and "intermediate" flags increased by 14.1%. In pediatric busulfan data, both "poor" and "intermediate" flagging decreased by 6.7% and 8.5%, respectively.
Conclusions: For MIPD solutions utilizing multiple published models, module-wide residual calculation improves the consistency of data flagging and reduces bias towards lenient models.
Aim: Evaluate the impact of using drug-module-wide error magnitude versus model-specific error magnitude on data flagging and model selection bias.
Methods: We explored mechanisms for flagging questionable data based on residuals (IWRES), comparing model-specific residual error with a constant residual error magnitude across all popPK models for a given drug. This was conducted in both adult vancomycin (N = 55,646) and pediatric busulfan (N = 650) patients from the InsightRX Nova platform where data are flagged as “good”, “intermediate”, or “poor” fit in relation to their calculated residual.
Results: Using model-specific residual error magnitude for flagging questionable observations may lead to user selection bias towards more lenient models, even if those models are less accurate for a patient. Switching to module-wide residual error magnitude reduced bias and provided more consistent flagging across stringent and lenient models. In adult vancomycin data, "poor" flags decreased by 16.9%, and "intermediate" flags increased by 14.1%. In pediatric busulfan data, both "poor" and "intermediate" flagging decreased by 6.7% and 8.5%, respectively.
Conclusions: For MIPD solutions utilizing multiple published models, module-wide residual calculation improves the consistency of data flagging and reduces bias towards lenient models.
Biography
Dr. Jordan Brooks, a Senior Pharmacometrician and Data Scientist at InsightRX, focuses on improving the predictive ability of precision dosing models and enhancing their usability for bedside clinicians. His work originated in pediatric hematopoietic cell transplantation with conditioning agents and immunosuppression, but now spans patients of all ages and various drug classes requiring precise dosing.
Dr Janice Goh
Scientist
Bioinformatics Institute, A*STAR
Optimizing doxorubicin dosing in Asian patients with ABCB1*13 polymorphisms
Abstract
Background: Doxorubicin is a narrow therapeutic index chemotherapeutic. ABCB1 is a major clearance pathway, making doxorubicin susceptible to variations in clearance due to pharmacogenomic (PGx) polymorphisms. However, pharmacogenomic clinical trials are resource intensive, making pharmacogenomic guided dose optimization difficult. Pharmacokinetic (PK) modelling and simulation tools help us evaluate drug-gene interaction (DGI) impact, and suggest optimized doses specific for Asian populations, saving resources.
Aims: We developed a PK model to evaluate doxorubicin-ABCB1 DGI, and suggest an optimized dose for ABCB1 polymorphisms.
Methods: Doxorubicin levels was simulated by merging information from a population PK (PPK) model and a noncompartmental analysis (NCA) pharmacogenomic PK study (NCA-informed PPK) to determine the impact of ABCB1*13 on drug exposure. 1000 simulations were run using Asian breast cancer demographics at a single intravenous dose of 60mg/m2.
Results: The NCA-informed PPK model was able to describe both wild type and ABCB1*13 changes in drug exposure per the NCA study. ABCB1*13 alone increased overall drug exposure (AUC) by 1.70 fold (1.38 – 2.03 fold, 95% confidence interval (CI)) compared to wild type ABCB1. Age > 60y/o with ABCB1*13 further increased AUC, with a 1.92 fold (1.58-2.30 fold, 95% CI) putting patients 13 at high risk of toxicity. Alternative dosing simulations suggest 35mg/m2 for people with ABCB1*13 polymorphisms.
Conclusions: The NCA-informed PPK model has helped us evaluate the impact of ABCB1*13 polymorphism on doxorubicin drug exposure and suggest new doses that might help patients with this polymorphism still benefit from the drug with lower risk of toxicity.
Keywords: Pharmacokinetics, pharmacogenomics
Aims: We developed a PK model to evaluate doxorubicin-ABCB1 DGI, and suggest an optimized dose for ABCB1 polymorphisms.
Methods: Doxorubicin levels was simulated by merging information from a population PK (PPK) model and a noncompartmental analysis (NCA) pharmacogenomic PK study (NCA-informed PPK) to determine the impact of ABCB1*13 on drug exposure. 1000 simulations were run using Asian breast cancer demographics at a single intravenous dose of 60mg/m2.
Results: The NCA-informed PPK model was able to describe both wild type and ABCB1*13 changes in drug exposure per the NCA study. ABCB1*13 alone increased overall drug exposure (AUC) by 1.70 fold (1.38 – 2.03 fold, 95% confidence interval (CI)) compared to wild type ABCB1. Age > 60y/o with ABCB1*13 further increased AUC, with a 1.92 fold (1.58-2.30 fold, 95% CI) putting patients 13 at high risk of toxicity. Alternative dosing simulations suggest 35mg/m2 for people with ABCB1*13 polymorphisms.
Conclusions: The NCA-informed PPK model has helped us evaluate the impact of ABCB1*13 polymorphism on doxorubicin drug exposure and suggest new doses that might help patients with this polymorphism still benefit from the drug with lower risk of toxicity.
Keywords: Pharmacokinetics, pharmacogenomics
Biography
Dr. Janice Goh graduated from NUS Pharmacy and is a registered pharmacist with the Singapore Pharmacy Council. She recently completed her PhD in the lab of Professor Rada Savic at the University of California, San Francisco (UCSF) School of Pharmacy. She joined the Research Data Integration group, Bioinformatics Institute as a Senior Scientist in Aug 2023. Her work focuses on utilizing pharmacokinetic and pharmacodynamic modeling and simulation tools to evaluate the impact of pharmacogenomics on drug disposition in real world settings.
Dr Kimitaka Suetsugu
Department of Pharmacy Kagoshima University Hospital
Machine Learning for Identifying Factors of Delayed Methotrexate Elimination in High-Dose Therapy
Abstract
Background: High-dose methotrexate (MTX) therapy is used for the treatment of gliomas, malignant lymphomas, acute lymphoblastic leukemia, and osteosarcoma. Nevertheless, delayed MTX elimination can occur, resulting in prolonged elevated plasma concentrations and severe adverse effects such as bone marrow suppression. The definitive factors contributing to this delay remain unclear.
Aims: This study aims to identify the factors influencing delayed MTX elimination through the application of machine learning techniques.
Methods: A total of 353 patients who received high-dose MTX at Kagoshima University Hospital between January 2015 and March 2021 were analyzed. Delayed elimination was defined as serum MTX levels of ≥0.1 μmol/L at 72 hours post-administration. Machine learning models were applied to analyze laboratory data and concomitant medication usage.
Results: Neural network analysis revealed that delayed elimination was not attributed to a single factor but rather to multiple interacting factors. The predictive accuracy was enhanced when patients with albumin measurements were selected. Moreover, the discontinuation of diuretics and NSAIDs in certain patients was associated with reduced MTX plasma concentrations.
Conclusions: Machine learning-based analysis suggests that early detection of delayed MTX elimination is feasible, which could lead to improved management of high-dose MTX therapy.
Key Words: High-dose methotrexate therapy, Delayed elimination, Machine Learning, Albumin
Aims: This study aims to identify the factors influencing delayed MTX elimination through the application of machine learning techniques.
Methods: A total of 353 patients who received high-dose MTX at Kagoshima University Hospital between January 2015 and March 2021 were analyzed. Delayed elimination was defined as serum MTX levels of ≥0.1 μmol/L at 72 hours post-administration. Machine learning models were applied to analyze laboratory data and concomitant medication usage.
Results: Neural network analysis revealed that delayed elimination was not attributed to a single factor but rather to multiple interacting factors. The predictive accuracy was enhanced when patients with albumin measurements were selected. Moreover, the discontinuation of diuretics and NSAIDs in certain patients was associated with reduced MTX plasma concentrations.
Conclusions: Machine learning-based analysis suggests that early detection of delayed MTX elimination is feasible, which could lead to improved management of high-dose MTX therapy.
Key Words: High-dose methotrexate therapy, Delayed elimination, Machine Learning, Albumin
Biography
Dr. Suetsugu earned a Ph.D. from Kyushu University. He is a hospital pharmacist engaged in Therapeutic Drug Monitoring (TDM). With extensive experience and dedication, Dr. Suetsugu has significantly contributed to optimizing pharmacotherapy and improving patient outcomes. His commitment to research and clinical practice has led to various publications and presentations at national and international conferences. Dr. Suetsugu remains passionate about advancing the field of clinical pharmacy and fostering collaborations among healthcare professionals to enhance patient care.
Mr Samuel Baroudi
Université de Rennes, CHU Rennes, Inserm, EHESP, IRSET-UMR S 1085. FHU SUPORT, Rennes, F-35000. Rennes, France
A new scoring methodology for implementation of POPPK models for TDM
Abstract
Background
Population pharmacokinetics (popPK) models are increasingly used in therapeutic drug monitoring. In some therapeutic areas, many models are available. Thus, selecting the most relevant model can be challenging, especially when no external evaluation dataset is available.
Aims
The objective was to develop a systematic scoring methodology to identify the most relevant popPK models and to showcase its use with tacrolimus models.
Methods
Mandatory inclusion/exclusion criteria were defined to exclude less relevant models and to focus on original models, reproducibility, and full PK profiles based-models. A generic scoring system based on literature guidelines, good practices in pharmacokinetic modeling and clinical relevance was developed. Then, a systematic review of the literature was conducted to identify candidate popPK models to be scored.
Results
Twenty-three criteria were selected. Dataset (patients/sample size), design (prospective, multicentric, analytical assay, data availability), methodology (methods/results of evaluation) and estimation of parameters (RSE%, residual error, shrinkage) had a score assigned based on weighted criteria.
Thirty-two (of 180) models were included in the final scoring process. Median score was 25/61 (range: 7-41), mean±SD was 24.65±7.77. Only 7/32 models scored above 30. Shortcomings included limited sampling, lack of external evaluation, missing covariates of interest, and limited methodological details.
Conclusions
This scoring system identifies best popPK models and is designed to be adaptable across different use-cases. This work also highlighted the heterogeneity and insufficiencies of reporting in popPK articles. Future work involves comparing these results to a statistical external evaluation to further validate the methodology.
Keyword
pharmacokinetics, external evaluation, scoring methodology.
Population pharmacokinetics (popPK) models are increasingly used in therapeutic drug monitoring. In some therapeutic areas, many models are available. Thus, selecting the most relevant model can be challenging, especially when no external evaluation dataset is available.
Aims
The objective was to develop a systematic scoring methodology to identify the most relevant popPK models and to showcase its use with tacrolimus models.
Methods
Mandatory inclusion/exclusion criteria were defined to exclude less relevant models and to focus on original models, reproducibility, and full PK profiles based-models. A generic scoring system based on literature guidelines, good practices in pharmacokinetic modeling and clinical relevance was developed. Then, a systematic review of the literature was conducted to identify candidate popPK models to be scored.
Results
Twenty-three criteria were selected. Dataset (patients/sample size), design (prospective, multicentric, analytical assay, data availability), methodology (methods/results of evaluation) and estimation of parameters (RSE%, residual error, shrinkage) had a score assigned based on weighted criteria.
Thirty-two (of 180) models were included in the final scoring process. Median score was 25/61 (range: 7-41), mean±SD was 24.65±7.77. Only 7/32 models scored above 30. Shortcomings included limited sampling, lack of external evaluation, missing covariates of interest, and limited methodological details.
Conclusions
This scoring system identifies best popPK models and is designed to be adaptable across different use-cases. This work also highlighted the heterogeneity and insufficiencies of reporting in popPK articles. Future work involves comparing these results to a statistical external evaluation to further validate the methodology.
Keyword
pharmacokinetics, external evaluation, scoring methodology.
Biography
n/a
Ms Woan Torng Tang
Universiti Sains Malaysia
Pharmacometrics-Based Time-to-Event Modelling of Major Bleeding in Atrial Fibrillation Patients on Rivaroxaban
Abstract
Background: The coexistence of atrial fibrillation (AF) and heart failure (HF) increases thromboembolic risk, with HF being an independent predictor of bleeding in AF patients on rivaroxaban.
Aims: This study aimed to develop a predictive model identifying factors affecting major bleeding risk, including HF severity markers linked to organ congestion, which may influence drug pharmacokinetics—serum albumin and right ventricular (RV) function.
Methods: Retrospective data from AF patients on rivaroxaban at the National Heart Institute, Malaysia, were analysed using pharmacometrics approaches, employing Nonlinear Mixed-Effect Modelling (NONMEM) software for parametric survival modelling. The model was validated via Kaplan-Meier visual predictive check (KM-VPC) and sampling-importance resampling (SIR).
Results: The final Weibull model indicated a decreasing hazard, with the highest bleeding risk early in treatment. Among 363 patients (876 observations), 32 experienced major bleeding over a follow-up of 1 day to 10.12 years (median 2.64, IQR 4.96 years). Higher serum albumin (>35 g/L) and tricuspid annular plane systolic excursion (TAPSE >1.8 cm) were protective against major bleeding, reducing hazard by 18.5% (aHR 0.81; 95% CI 0.78–0.86) and 81.4% (aHR 0.19; 95% CI 0.08–0.43), respectively. Aspirin (aHR 5.41; 95% CI 2.19–13.39), dual antiplatelet therapy (aHR 2.09; 95% CI 1.07–4.09), clopidogrel (aHR 1.72; 95% CI 1.07–2.78), and hypertension (aHR 5.57; 95% CI 1.09–28.33) increased bleeding risk.
Conclusions: This study highlights the protective effects of serum albumin and RV function in rivaroxaban users. Given rivaroxaban’s high protein binding and hepatic metabolism, it offers insights into HF-related hypoalbuminaemia and hepatic congestion from RV dysfunction in anticoagulation management.
Aims: This study aimed to develop a predictive model identifying factors affecting major bleeding risk, including HF severity markers linked to organ congestion, which may influence drug pharmacokinetics—serum albumin and right ventricular (RV) function.
Methods: Retrospective data from AF patients on rivaroxaban at the National Heart Institute, Malaysia, were analysed using pharmacometrics approaches, employing Nonlinear Mixed-Effect Modelling (NONMEM) software for parametric survival modelling. The model was validated via Kaplan-Meier visual predictive check (KM-VPC) and sampling-importance resampling (SIR).
Results: The final Weibull model indicated a decreasing hazard, with the highest bleeding risk early in treatment. Among 363 patients (876 observations), 32 experienced major bleeding over a follow-up of 1 day to 10.12 years (median 2.64, IQR 4.96 years). Higher serum albumin (>35 g/L) and tricuspid annular plane systolic excursion (TAPSE >1.8 cm) were protective against major bleeding, reducing hazard by 18.5% (aHR 0.81; 95% CI 0.78–0.86) and 81.4% (aHR 0.19; 95% CI 0.08–0.43), respectively. Aspirin (aHR 5.41; 95% CI 2.19–13.39), dual antiplatelet therapy (aHR 2.09; 95% CI 1.07–4.09), clopidogrel (aHR 1.72; 95% CI 1.07–2.78), and hypertension (aHR 5.57; 95% CI 1.09–28.33) increased bleeding risk.
Conclusions: This study highlights the protective effects of serum albumin and RV function in rivaroxaban users. Given rivaroxaban’s high protein binding and hepatic metabolism, it offers insights into HF-related hypoalbuminaemia and hepatic congestion from RV dysfunction in anticoagulation management.
Biography
She is a final-year PhD student at Universiti Sains Malaysia, under the supervision of Dr. Siti Maisharah Sheikh Ghadzi and Dr. Sabariah Noor Harun. Her research focuses on the population pharmacokinetics and pharmacodynamics of rivaroxaban in patients with atrial fibrillation, with a particular emphasis on the relatively under-researched heart failure population in pharmacokinetics. Her study was conducted at Institut Jantung Negara (IJN), a leading cardiovascular care centre in Malaysia, and was supported by a grant from the IJN Foundation. Prior to pursuing her PhD, she gained extensive experience in managing pharmacotherapy for a wide range of medical and surgical cases at government tertiary hospitals.
Dr Julian Otalvaro
2. Laboratory of Applied Pharmacokinetics and Bioinformatics, Children’s Hospital Los Angeles, Keck School of Medicine
Stochastic Differential Equations for Modeling Nonparametric Inter-Occasion Variability (IOV) in Pharmacokinetics (PK)
Abstract
Background: PK IOV is typically modeled as a stepwise random parameter based on sampling occasions using Ordinary Differential Equation (ODE) solvers. In contrast, SDEs allow more physiologic, continuously time-dependent PK parameters. However, due to computational complexity, SDEs are rarely used in parametric popPK modeling and have not been implemented in a nonparametric framework.
Aims: Develop a practical nonparametric SDE popPK solver.
Methods
We implemented a custom SDE solver with particle filtering in Pmetrics. From this, we simulated 4 PK parameter datasets for a 1-compartment IV model (Ke, V) with/without inter-individual variability (IIV) and/or IOV. All datasets comprised 100 subjects. We compared SDE fits to true simulated values.
Results:
# CONDITIONS TRUE FITTED
Mean SD IOV Mean SD IOV
----------------------------------------------------------------
1 Ke +IIV, -IOV 0.96 0.5 0% 1.16 0.47 0
V fixed
2 Ke +IIV, +IOV 0.96 0.5 104% 1.07 0.51 63%
V fixed
3 Ke +IIV, -IOV 0.96 0.5 0% 1.08 0.50 0
V +IIV, -IOV 1.04 0.2 0% 1.05 0.20 0
4 Ke +IIV, +IOV 0.96 0.5 104% 0.97 0.56 108%
V +IIV, +IOV 1.04 0.2 96% 1.07 0.21 85%
As further validation for #1 (no IOV), with ODE we found mean (SD) Ke of 1.23 (0.5196) and the same likelihood of 920.76 that we obtained for SDE.
Conclusions: SDEs with particle filtering provide a robust framework for modeling nonparametric IOV in pharmacokinetics. This approach accurately estimates parameter values in the presence of continuous IOV and may enhance individualized dosing strategies.
Keywords: pharmacokinetics, nonparametric methods, inter-occasion variability, parameter evolution
Aims: Develop a practical nonparametric SDE popPK solver.
Methods
We implemented a custom SDE solver with particle filtering in Pmetrics. From this, we simulated 4 PK parameter datasets for a 1-compartment IV model (Ke, V) with/without inter-individual variability (IIV) and/or IOV. All datasets comprised 100 subjects. We compared SDE fits to true simulated values.
Results:
# CONDITIONS TRUE FITTED
Mean SD IOV Mean SD IOV
----------------------------------------------------------------
1 Ke +IIV, -IOV 0.96 0.5 0% 1.16 0.47 0
V fixed
2 Ke +IIV, +IOV 0.96 0.5 104% 1.07 0.51 63%
V fixed
3 Ke +IIV, -IOV 0.96 0.5 0% 1.08 0.50 0
V +IIV, -IOV 1.04 0.2 0% 1.05 0.20 0
4 Ke +IIV, +IOV 0.96 0.5 104% 0.97 0.56 108%
V +IIV, +IOV 1.04 0.2 96% 1.07 0.21 85%
As further validation for #1 (no IOV), with ODE we found mean (SD) Ke of 1.23 (0.5196) and the same likelihood of 920.76 that we obtained for SDE.
Conclusions: SDEs with particle filtering provide a robust framework for modeling nonparametric IOV in pharmacokinetics. This approach accurately estimates parameter values in the presence of continuous IOV and may enhance individualized dosing strategies.
Keywords: pharmacokinetics, nonparametric methods, inter-occasion variability, parameter evolution
Biography
Julián David Otálvaro currently works at the Laboratory of Applied Pharmacokinetics and Bioinformatics - CHLA/USC. Under the supervision of Michael Neely and Walter Yamada He is currently working on further ways of integrate pharmacometric and machine learning methods.
Ms 女士 Jing Wang
Nanjing Drum Tower Hospital
Population Pharmacokinetics and Dose Optimization of Voriconazole in COVID-19-Associated Pulmonary Aspergillosis Patients
Abstract
Background: Under the backdrop of the COVID-19 pandemic, the incidence of COVID-19-associated pulmonary aspergillosis (CAPA) has progressively increased. Voriconazole, as a first-line therapeutic agent, has been widely utilized in the management of this condition. However, due to its nonlinear pharmacokinetics, voriconazole exhibits significant interindividual variability in plasma concentrations. This necessitates the implementation of precision dosing strategies to optimize therapeutic efficacy while minimizing adverse drug reactions.
Aims: The study aimed to investigate the pharmacokinetic profile of voriconazole in CAPA patients to optimize dosing strategies.
Methods: Population pharmacokinetic modeling was conducted using clinical data from CAPA patients to analyze voriconazole's pharmacokinetic behavior. A one-compartment model with first-order absorption and elimination was employed to characterize voriconazole disposition. Covariate analysis was further utilized to evaluate the impact of continuous renal replacement therapy (CRRT) and select biochemical markers on voriconazole clearance.
Results: The model estimated voriconazole's apparent clearance (CL/F) at 3.17 L/h and volume of distribution (V/F) at 135 L for a standard patient with CAPA. Covariates including CRRT, C-reactive protein, gamma-glutamyl transpeptidase, aspartate aminotransferase, and platelet count were found to significantly influence voriconazole clearance. Monte Carlo simulations indicated that CRRT patients required both a higher loading dose and an increased maintenance dose compared to those not on CRRT.
Conclusions: This study provides an evidence-based guide for voriconazole dosing adjustments in CAPA patients, particularly for those undergoing CRRT. The findings emphasize the importance of individualized dosing to improve therapeutic outcomes in this high-risk population.
Keywords: COVID-19-Associated Pulmonary Aspergillosis; voriconazole; population pharmacokinetics; dosage optimization; monte carlo
Aims: The study aimed to investigate the pharmacokinetic profile of voriconazole in CAPA patients to optimize dosing strategies.
Methods: Population pharmacokinetic modeling was conducted using clinical data from CAPA patients to analyze voriconazole's pharmacokinetic behavior. A one-compartment model with first-order absorption and elimination was employed to characterize voriconazole disposition. Covariate analysis was further utilized to evaluate the impact of continuous renal replacement therapy (CRRT) and select biochemical markers on voriconazole clearance.
Results: The model estimated voriconazole's apparent clearance (CL/F) at 3.17 L/h and volume of distribution (V/F) at 135 L for a standard patient with CAPA. Covariates including CRRT, C-reactive protein, gamma-glutamyl transpeptidase, aspartate aminotransferase, and platelet count were found to significantly influence voriconazole clearance. Monte Carlo simulations indicated that CRRT patients required both a higher loading dose and an increased maintenance dose compared to those not on CRRT.
Conclusions: This study provides an evidence-based guide for voriconazole dosing adjustments in CAPA patients, particularly for those undergoing CRRT. The findings emphasize the importance of individualized dosing to improve therapeutic outcomes in this high-risk population.
Keywords: COVID-19-Associated Pulmonary Aspergillosis; voriconazole; population pharmacokinetics; dosage optimization; monte carlo
Biography
Jing Wang holds a Master's degree in Pharmacy and is employed at Nanjing Drum Tower Hospital, a well-known medical institution in China. Her research primarily focuses on drug analysis and population pharmacokinetics, contributing to the understanding of drug behavior in various patient groups.
As a first author, Jing has published five articles in SCI-indexed journals and two papers in Chinese core journals. Her work demonstrates her involvement in pharmaceutical research and her contributions to the field.
Mrs Rao Du
Children's Hospital Affiliated To Soochow University
Predicting Tacrolimus Blood Concentration in Children Undergoing HSCT Using Machine Learning Algorithms
Abstract
Background: Tacrolimus has a narrow therapeutic window and significant individual differences. The blood concentration of tacrolimus in children undergoing hematopoietic cell transplantation (HSCT) cannot be controlled within the effective concentration range, which will seriously affect the prognosis of the children.
Aims: To construct a predictive model for the factors influencing tacrolimus blood concentration in HSCT children using machine learning algorithms.
Methods: The treatment data of HSCT children of the Children's Hospital of Soochow University in 2024 were collected. Eight machine learning algorithms: logistic regression, logistic regression + minimum absolute contraction and selection operator, decision tree, random forest, extreme Gradient boost, gradient Boost, CatBoost gradient boost, K-nearest neighbor were used to construct the prediction model. The area under the receiver operation characteristic curve (AUC), accuracy rate, recall rate, accuracy rate and F1 score were used as comparison indexes among different models, and the CatBoost model was optimized by random search.
Results: A total of 346 cases of HSCT children were included. According to the AUC, the CatBoost model showed the best predictive performance for tacrolimus concentration in both the internal and external validation cohorts. The internal validation cohort had an AUC of 0.93 [95% CI: 0.90-0.95], and the external validation cohort had an AUC of 0.91 [95% CI: 0.89-0.94]. Genotype, serum albumin and age were most important predictors of tacrolimus blood concentration.
Conclusion: This study developed a predictive model based on machine learning algorithms, which can predict the concentration range of tacrolimus in HSCT children.
Keywords: tacrolimus, blood concentration, machine learning
Aims: To construct a predictive model for the factors influencing tacrolimus blood concentration in HSCT children using machine learning algorithms.
Methods: The treatment data of HSCT children of the Children's Hospital of Soochow University in 2024 were collected. Eight machine learning algorithms: logistic regression, logistic regression + minimum absolute contraction and selection operator, decision tree, random forest, extreme Gradient boost, gradient Boost, CatBoost gradient boost, K-nearest neighbor were used to construct the prediction model. The area under the receiver operation characteristic curve (AUC), accuracy rate, recall rate, accuracy rate and F1 score were used as comparison indexes among different models, and the CatBoost model was optimized by random search.
Results: A total of 346 cases of HSCT children were included. According to the AUC, the CatBoost model showed the best predictive performance for tacrolimus concentration in both the internal and external validation cohorts. The internal validation cohort had an AUC of 0.93 [95% CI: 0.90-0.95], and the external validation cohort had an AUC of 0.91 [95% CI: 0.89-0.94]. Genotype, serum albumin and age were most important predictors of tacrolimus blood concentration.
Conclusion: This study developed a predictive model based on machine learning algorithms, which can predict the concentration range of tacrolimus in HSCT children.
Keywords: tacrolimus, blood concentration, machine learning
Biography
Rao DU ,Chief Pharmacist, Master of Pharmacology.Currently serving as the Deputy Director of the Pharmacy Department and Clinical Pharmacist of the Pediatric Hematology Department at Suzhou University Affiliated Children's Hospital.
Mr Michael Stokes
Schn And University Of Sydney
A Scoping review of the Paediatric Population Pharmacokinetic Models of Morphine
Abstract
Background
Morphine dosing in paediatrics is dependent on imprecise measures of pain and clinician experience. Multiple population pharmacokinetic models have been developed to identify and quantify factors contributing to variability in morphine pharmacokinetics with the aim of guiding dosing decisions.
Aim
To summarise available population pharmacokinetic models of morphine and its metabolites (M3G and M6G) in paediatric patients to describe how morphine exposure changes across paediatric age groups.
Methods
EMBASE and MEDLINE databases were systematically searched from inception to 8th March 2024 for articles containing paediatric population pharmacokinetic models of morphine and its metabolites. Two independent reviewers (MS and NK) screened abstracts, full texts, and extracted data, which was then summarised. The review was conducted according to PRISMA scoping review guidelines.
Results
Overall, 21 paediatric population pharmacokinetic models of morphine were identified, with 12 studies including morphine metabolite (M3G and/or M6G) pharmacokinetics. Neonates and young children (< 6 years) were the most studied age groups (18/21; 86%), while older children (> 6 years) and adolescents were included in only 6/21 (29%) models. Age, post-natal age for neonates, and bodyweight were found to be significant covariates for morphine clearance. Additional covariates relating to body temperature, duration-of-stay, and genetic variation in drug transporters which mediate the uptake of morphine (e.g. OCT1) were also identified.
Conclusion
Several population pharmacokinetic models of morphine and its metabolites in the paediatric population exist, but the influence of organ dysfunction, atypical disease states, including critical illness, and the effect of long-term treatment on morphine pharmacokinetics remains underexplored.
Morphine dosing in paediatrics is dependent on imprecise measures of pain and clinician experience. Multiple population pharmacokinetic models have been developed to identify and quantify factors contributing to variability in morphine pharmacokinetics with the aim of guiding dosing decisions.
Aim
To summarise available population pharmacokinetic models of morphine and its metabolites (M3G and M6G) in paediatric patients to describe how morphine exposure changes across paediatric age groups.
Methods
EMBASE and MEDLINE databases were systematically searched from inception to 8th March 2024 for articles containing paediatric population pharmacokinetic models of morphine and its metabolites. Two independent reviewers (MS and NK) screened abstracts, full texts, and extracted data, which was then summarised. The review was conducted according to PRISMA scoping review guidelines.
Results
Overall, 21 paediatric population pharmacokinetic models of morphine were identified, with 12 studies including morphine metabolite (M3G and/or M6G) pharmacokinetics. Neonates and young children (< 6 years) were the most studied age groups (18/21; 86%), while older children (> 6 years) and adolescents were included in only 6/21 (29%) models. Age, post-natal age for neonates, and bodyweight were found to be significant covariates for morphine clearance. Additional covariates relating to body temperature, duration-of-stay, and genetic variation in drug transporters which mediate the uptake of morphine (e.g. OCT1) were also identified.
Conclusion
Several population pharmacokinetic models of morphine and its metabolites in the paediatric population exist, but the influence of organ dysfunction, atypical disease states, including critical illness, and the effect of long-term treatment on morphine pharmacokinetics remains underexplored.
Biography
Michael is a senior Pharmacist in the Paediatric Palliative Care service for the Sydney Children's Hospitals Network. He has substantial experience in Paediatric Intensive Care medicines and is a PhD candidate under the supervision of Assoc. Prof. Sophie Stocker.
Assoc Prof Hesham Al-Sallami
College of Pharmacy, QU Health, Qatar University
Individualising insulin bolus dosing in children diagnosed with type 1 diabetes mellitus
Abstract
Background: Insulin dose requirements vary among patients with type 1 diabetes mellitus (T1DM). Bolus doses are typically determined by a patient’s insulin to carbohydrate ratio (ICR), for meal-time dosing, and the correction factor (CF), for elevated blood-glucose correction. Accurately determining a patient’s ICR and CF values often requires several days of iterative dose adjustments, guided by blood glucose monitoring and carbohydrate counting.
Aims: To develop and evaluate predictive models of ICR and CF to improve insulin dosing strategies in newly diagnosed paediatric T1DM patients.
Methods: A retrospective analysis of clinical, laboratory, and insulin dosing data was conducted on 70 children (≤ 18 years old) hospitalised for newly diagnosed T1DM. Linear regression models were developed to predict ICR and CF based on clinical characteristics, with model accuracy assessed using mean error (bias) and root mean squared error (precision).
Results: Based on the developed ICR and CF prediction models, for patients < 6 years old, total daily dose of insulin administered on first day (TDD_first) was the only significant predictor for ICR and CF (p < 0.05).
For patients ≥ 6 years old, patient’s TDD_first and age were found to be significant predictors for both ICR and CF (p < 0.05).
Conclusion: Accounting for a patient’s age and initial insulin total daily dose allows for better estimation of true (i.e. asymptotic) ICR and CF values. This can potentially provide better glycaemic control sooner during hospital stay. These findings suggest a data-driven approach to personalising insulin therapy in newly diagnosed paediatric T1DM patients.
Aims: To develop and evaluate predictive models of ICR and CF to improve insulin dosing strategies in newly diagnosed paediatric T1DM patients.
Methods: A retrospective analysis of clinical, laboratory, and insulin dosing data was conducted on 70 children (≤ 18 years old) hospitalised for newly diagnosed T1DM. Linear regression models were developed to predict ICR and CF based on clinical characteristics, with model accuracy assessed using mean error (bias) and root mean squared error (precision).
Results: Based on the developed ICR and CF prediction models, for patients < 6 years old, total daily dose of insulin administered on first day (TDD_first) was the only significant predictor for ICR and CF (p < 0.05).
For patients ≥ 6 years old, patient’s TDD_first and age were found to be significant predictors for both ICR and CF (p < 0.05).
Conclusion: Accounting for a patient’s age and initial insulin total daily dose allows for better estimation of true (i.e. asymptotic) ICR and CF values. This can potentially provide better glycaemic control sooner during hospital stay. These findings suggest a data-driven approach to personalising insulin therapy in newly diagnosed paediatric T1DM patients.
Biography
Hesham Al-Sallami is an associate professor of clinical pharmacy practice. He holds a PhD (University of Otago) in quantitative clinical pharmacology and pharmacometrics. Hesham's research interests include drug dose individualisation in special populations, with a focus on paediatrics and in obesity.
Assoc Prof Jeffrey Pradeep Raj
Kasturba Medical College Manipal, Manipal Academy of Higher Education
Assessment of Levetiracetam Therapeutic Drug Monitoring data using Pharmacometric Approach.
Abstract
Background: Levetiracetam is an anti-epileptic agent that has been approved for use in partial-onset, myoclonic, primarily generalized tonic-clonic seizures as adjunctive therapy. The
recommended therapeutic range is 12-46 mg/L.
Aim: To perform a population pharmacokinetics (Pop-PK) analysis in adult patients from India.
Methods: Blood samples from n=245 patients, analyzed for drug concentrations as part of routine clinical care, were included in the analysis. As all were trough samples, and Pop-PK modeling requires varied time points, we tried to adapt a suitable model from the existing models in literature.
Results: The Toublanc et al. model developed in the Japanese population and tested in the North American population was able to predict the time versus concentration profile in our study population. It is a one-compartment model with body weight, the volume of distribution, and enzyme-inducing agents as covariates on clearance. Weight was normalized to 32 with the exponents of 0.75 and 1 for clearance and volume of distribution, respectively. We did not use the enzyme inducer as a covariate as observed scatter data was successfully overlapped on simulated plots for each dosage regimen by using weight alone as a covariate. Random variability on CL, V, and Ka were 20%, 12.24%, and 85%, respectively, and residual variability was 20% based on published literature.
Conclusions: Thus, we conclude that the Toublanc et al. model may suit our study population and plan to validate it by obtaining additional samples at different time points to develop a population-specific model.
recommended therapeutic range is 12-46 mg/L.
Aim: To perform a population pharmacokinetics (Pop-PK) analysis in adult patients from India.
Methods: Blood samples from n=245 patients, analyzed for drug concentrations as part of routine clinical care, were included in the analysis. As all were trough samples, and Pop-PK modeling requires varied time points, we tried to adapt a suitable model from the existing models in literature.
Results: The Toublanc et al. model developed in the Japanese population and tested in the North American population was able to predict the time versus concentration profile in our study population. It is a one-compartment model with body weight, the volume of distribution, and enzyme-inducing agents as covariates on clearance. Weight was normalized to 32 with the exponents of 0.75 and 1 for clearance and volume of distribution, respectively. We did not use the enzyme inducer as a covariate as observed scatter data was successfully overlapped on simulated plots for each dosage regimen by using weight alone as a covariate. Random variability on CL, V, and Ka were 20%, 12.24%, and 85%, respectively, and residual variability was 20% based on published literature.
Conclusions: Thus, we conclude that the Toublanc et al. model may suit our study population and plan to validate it by obtaining additional samples at different time points to develop a population-specific model.
Biography
Dr. Jeffrey Pradeep Raj is an Associate Professor in the Division of Clinical Pharmacology at Kasturba Medical College, Manipal Academy of Higher Education (MAHE), India. A gold medalist in DM Clinical Pharmacology from Seth GS Medical College & KEM Hospital, Mumbai, and MD Pharmacology from St. John’s Medical College, Bengaluru, he has received numerous academic accolades. His research expertise spans clinical trials, pharmacokinetics, and vaccine acceptance studies. He has authored multiple peer-reviewed publications and serves on the editorial boards of international journals. Dr. Raj has been a lead investigator in several sponsored clinical trials and has delivered invited talks at global conferences. Actively involved in medical education and ethics, he has mentored MBBS and MD students and contributed to policy discussions. His interdisciplinary interests include biostatistics, health economics, precision medicine and drug safety, making him a key contributor to clinical pharmacology research in India.
Mr Lu Jin
Department Of Pharmacy,the Affiliated Drum Tower Hospital Of Nanjing University Medical School
Evaluation of Tigecycline Dosing Regimen for Patients Using Monte Carlo Simulation
Abstract
Background: Monte Carlo simulation using population pharmacokinetic parameters of patient is a commonly used method to evaluate the effectiveness of antimicrobial drugs, which is mostly used to compare the bactericidal effect of different dosing regimens against a particular bacterium.
Aims: Monte Carlo simulation was applied to explore the optimal dosage regimen of tigecycline when applied to patients with severe infections in combination with reported population pharmacokinetic models .
Methods: Four population pharmacokinetic models were retrieved and selected, covering cIAI, cSSSI, CAP and MDR infected patient populations. Monte Carlo simulation was performed to simulate the AUC0-24 values at different administered doses, and probability of target attainment analyses were performed in conjunction with efficacy and safety thresholds. This was followed by comparative validation using clinical data from our institution.
Results: Simulation results on each model showed that the high dose (initial dose of 200 mg, maintenance of 100 mg, q12h) of tigecycline compared to the standard dose (initial dose of 100 mg, maintenance of 50 mg, q12h) increased the risk of hypofibrinogenaemia while improving efficacy. Clinical validation data showed that AUC0-24 was significantly higher in the high-dose group than in the standard-dose group, but the incidence of adverse events also increased.
Conclusions: Clinicians need to pay close attention to the hypofibrinogenaemia caused by high-dose tigecycline when applying it to ICU patients, and regular therapeutic drug monitoring is recommended during treatment to ensure safe and effective use of the drug.
Key Words: Tigecycline; Monte Carlo simulation; Pharmacokinetic/pharmacodynamic modelling; Therapeutic drug monitoring
Aims: Monte Carlo simulation was applied to explore the optimal dosage regimen of tigecycline when applied to patients with severe infections in combination with reported population pharmacokinetic models .
Methods: Four population pharmacokinetic models were retrieved and selected, covering cIAI, cSSSI, CAP and MDR infected patient populations. Monte Carlo simulation was performed to simulate the AUC0-24 values at different administered doses, and probability of target attainment analyses were performed in conjunction with efficacy and safety thresholds. This was followed by comparative validation using clinical data from our institution.
Results: Simulation results on each model showed that the high dose (initial dose of 200 mg, maintenance of 100 mg, q12h) of tigecycline compared to the standard dose (initial dose of 100 mg, maintenance of 50 mg, q12h) increased the risk of hypofibrinogenaemia while improving efficacy. Clinical validation data showed that AUC0-24 was significantly higher in the high-dose group than in the standard-dose group, but the incidence of adverse events also increased.
Conclusions: Clinicians need to pay close attention to the hypofibrinogenaemia caused by high-dose tigecycline when applying it to ICU patients, and regular therapeutic drug monitoring is recommended during treatment to ensure safe and effective use of the drug.
Key Words: Tigecycline; Monte Carlo simulation; Pharmacokinetic/pharmacodynamic modelling; Therapeutic drug monitoring
Biography
Deputy Chief Pharmacist, Nanjing DrumTower Hospital, China. Mainly engaged in therapeutic drug monitoring. Research area is pharmacokinetics/pharmacodynamics of antimicrobial drugs.
Mr Jie Cao
Hangzhou Red Cross Hospital
Population Pharmacokinetics of Cycloserine: A Systematic Review
Abstract
Background: Cycloserine (CS) plays a key role in the treatment of multi-drug resistant tuberculosis. However, limited pharmacokinetic (PK) data on cycloserine in TB patients and individual differences in drug use have led to unsatisfactory therapeutic outcomes and uncontrolled adverse events.
Aims: To review the published PK models of CS and identify important covariates affecting CS PK differences by comparing the published CS population pharmacokinetic models, so as to provide guidance for clinical drug use.
Methods: A systematic search was made of PubMed and Web of Science databases from establishment to December 2024 on the pharmacokinetics of CS populations. The PK characteristics and significant covariates included in the study were systematically summarized.
Results: Six studies that met the criteria were included in our review. These studies were all conducted in adults, and the apparent clearance and volume of distribution of CS to homeostasis ranged from 0.75 to 3.51 L/h and 4.86 to 34.56 L, respectively. Weight and creatinine clearance were important covariates affecting clearance, with CL/F estimates (2.00 L/h) about twice as high in healthy subjects as in TB patients (1.03 L/h) and smoking increasing CS clearance by 41%.
Conclusions: This article reviews the covariates that may be responsible for individual differences among patients taking CS, which is helpful to provide clinical recommendations for doctors. However, further research is needed to verify the effects of these covariates and to explore other possible covariates.
Keywords:cycloserine, multidrug-resistant tuberculosis, population pharmacokinetics, nonlinear mixed-effect modeling
Aims: To review the published PK models of CS and identify important covariates affecting CS PK differences by comparing the published CS population pharmacokinetic models, so as to provide guidance for clinical drug use.
Methods: A systematic search was made of PubMed and Web of Science databases from establishment to December 2024 on the pharmacokinetics of CS populations. The PK characteristics and significant covariates included in the study were systematically summarized.
Results: Six studies that met the criteria were included in our review. These studies were all conducted in adults, and the apparent clearance and volume of distribution of CS to homeostasis ranged from 0.75 to 3.51 L/h and 4.86 to 34.56 L, respectively. Weight and creatinine clearance were important covariates affecting clearance, with CL/F estimates (2.00 L/h) about twice as high in healthy subjects as in TB patients (1.03 L/h) and smoking increasing CS clearance by 41%.
Conclusions: This article reviews the covariates that may be responsible for individual differences among patients taking CS, which is helpful to provide clinical recommendations for doctors. However, further research is needed to verify the effects of these covariates and to explore other possible covariates.
Keywords:cycloserine, multidrug-resistant tuberculosis, population pharmacokinetics, nonlinear mixed-effect modeling
Biography
a clinical pharmacy worker
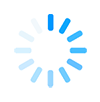