SYMPOSIUM 12: Personalised dosing at the bedside: Model selection versus model averaging
Tracks
Track 4
Tuesday, September 23, 2025 |
10:30 AM - 12:00 PM |
Grand Copthorne Waterfront Hotel - Waterfront Ballroom III |
Details
Model-based dosing requires a suitably predictive model. Often, multiple models are available for a given drug, and so the clinician is faced with a decision of how to best treat their patient. In recent years, two approaches have been proposed: (i) model selection, in which one model of several is selected for a given patient and used to inform dose selection, and (ii) model averaging, in which the predictions from multiple models are combined to inform dose selection.
Both model selection and model averaging can be implemented in different ways, ranging from statistical approaches to machine learning algorithms. These approaches come with clinical implementation trade-offs, regulatory differences, and differences in predictive performance. In this symposium, considerations for each approach are explored and compared.
Speaker
Dr Jasmine Hughes
Director Of Data Science
Insightrx
From big data to better dosing: Optimizing patient-specific PK model selection
Abstract
Background
Successful model-informed precision dosing (MIPD) depends on the ability of the model to describe the patient. However, models may not fully capture the diversity of patients, with some models being more predictive in certain subpopulations than others. In current practice, model selection is often manual, based on simplistic rules (e.g., BMI cutoffs), or defaulted to a single model without empirical validation across diverse cohorts.
Aims
We developed a data-driven, pre-TDM model selection algorithm guided by expert-derived design principles and grounded in real-world experience supporting vancomycin dosing.
Methods
We defined seven principles to guide model selection: (1) evaluate models as used in clinical workflows (stepwise, without future data); (2) emphasize a priori predictive performance; (3) favor models that minimize the risk of underexposure; (4) prefer models developed in clinically similar populations; (5) reflect known drug PK structure (e.g., two-compartment models); (6) incorporate clinical implementation experience; and (7) optimize based on error magnitude, bias, and prediction accuracy. Seven vancomycin PK models were evaluated across 129 patient archetypes defined by age, renal function, BMI, and sex, in a data set of 384,000 treatment courses.
Results
Evaluated in a 145,000-patient hold-out cohort, the algorithm reduced bias by 85–88%, improved prediction accuracy by up to 50%, and lowered RMSE by 10–22% compared to general-use, single-model approaches.
Conclusions
Expert-informed, data-backed model selection improves predictive performance while maintaining transparency required for clinical adoption. Future work may explore integrating machine learning methods capable of leveraging high-dimensional EHR data to further refine model selection, particularly for complex or underrepresented subpopulations.
Successful model-informed precision dosing (MIPD) depends on the ability of the model to describe the patient. However, models may not fully capture the diversity of patients, with some models being more predictive in certain subpopulations than others. In current practice, model selection is often manual, based on simplistic rules (e.g., BMI cutoffs), or defaulted to a single model without empirical validation across diverse cohorts.
Aims
We developed a data-driven, pre-TDM model selection algorithm guided by expert-derived design principles and grounded in real-world experience supporting vancomycin dosing.
Methods
We defined seven principles to guide model selection: (1) evaluate models as used in clinical workflows (stepwise, without future data); (2) emphasize a priori predictive performance; (3) favor models that minimize the risk of underexposure; (4) prefer models developed in clinically similar populations; (5) reflect known drug PK structure (e.g., two-compartment models); (6) incorporate clinical implementation experience; and (7) optimize based on error magnitude, bias, and prediction accuracy. Seven vancomycin PK models were evaluated across 129 patient archetypes defined by age, renal function, BMI, and sex, in a data set of 384,000 treatment courses.
Results
Evaluated in a 145,000-patient hold-out cohort, the algorithm reduced bias by 85–88%, improved prediction accuracy by up to 50%, and lowered RMSE by 10–22% compared to general-use, single-model approaches.
Conclusions
Expert-informed, data-backed model selection improves predictive performance while maintaining transparency required for clinical adoption. Future work may explore integrating machine learning methods capable of leveraging high-dimensional EHR data to further refine model selection, particularly for complex or underrepresented subpopulations.
Biography
Dr Jasmine Hughes is the Head of Clinical Data Science at InsightRX, a San Francisco-based company that makes model-informed precision dosing and clinical analytics software. She received her Bachelor's in Chemical Engineering from McGill University and her PhD in Bioengineering from the University of California, San Francisco and the University of California, Berkeley, and is currently based in Toronto, Canada. Her current research focus is creating and improving models, statistical methods and software tools for improving dose individualization in clinical practice.
Prof Sebastian Wicha
University of Hamburg
Does it need a team to win? Model averaging in model-informed precision dosing
Abstract
Model-informed precision dosing (MIPD) integrates population pharmacokinetic (PK) models with drug concentration data obtained through therapeutic drug monitoring to optimize individualized dosing. A critical determinant of accurate and precise predictions is the selection of an appropriate population PK model—ideally, one that closely matches the patient’s characteristics. However, identifying the most suitable model for a given patient can be challenging in routine clinical practice.
Model averaging has been proposed as a strategy to address this challenge. Rather than relying on a single model, model averaging incorporates multiple candidate models, estimates the individual pharmacokinetic profile using each, and then computes a weighted average of the forecasts based on model performance metrics.
This presentation will begin with an overview of the principles of model averaging and its application in clinical pharmacology. We will review and critically evaluate the current literature on the use of model averaging in MIPD. Finally, we will present findings from a simulation study conducted by our research group, offering practical insights into the selection of a meaningful set of candidate models for model averaging.
Model averaging has been proposed as a strategy to address this challenge. Rather than relying on a single model, model averaging incorporates multiple candidate models, estimates the individual pharmacokinetic profile using each, and then computes a weighted average of the forecasts based on model performance metrics.
This presentation will begin with an overview of the principles of model averaging and its application in clinical pharmacology. We will review and critically evaluate the current literature on the use of model averaging in MIPD. Finally, we will present findings from a simulation study conducted by our research group, offering practical insights into the selection of a meaningful set of candidate models for model averaging.
Biography
Sebastian Wicha is a tenured Full Professor of Clinical Pharmacy. He is leading the research group Clinical Pharmacy at the Institute of Pharmacy, University of Hamburg, Germany since 2017. He is a pharmacist by training holding a Ph.D. in Clinical Pharmacy from the Freie Universität Berlin, Germany (2015) and gained experience as a postdoctoral researcher at the Pharmacometrics Research Group at Uppsala University, Sweden (2015-2017). His research group aims to improve and individualize drug therapy by means of pharmacokinetic-pharmacodynamic and pharmacometric techniques with a special focus on anti-infectives. In this field, he has published >110 peer-reviewed papers. His research group has expertise in bioanalysis, pharmacometric data analysis, and develops open-access web-based software to foster model-informed precision dosing (TDMx.eu). Sebastian is the currently the chairperson of the PK/PD study group of the European Society for Clinical Microbiology and Infectious Diseases (ESCMID) as well as an ESCMID Fellow. Moreover, he is an external expert for pharmacometrics for the German Federal Institute for Drugs and Medical Devices (BfArM) and the European Medicines Agency (EMA).
Dr Nynke Jager
Clinical Pharmacist
Radboudumc
Using models in clinical practice – How to get there through retrospective and prospective evaluations
Abstract
Model-Informed Precision Dosing (MIPD) is increasingly recognized as a valuable tool for enhancing individualized pharmacotherapy. By combining mathematical pharmacological information on drugs and diseases (i.e. population pharmacokinetic models) with patient-specific clinical data, MIPD enables more precise dosing decisions in routine clinical practice.
Despite its potential, widespread adoption of MIPD in clinical settings remains limited. Before this approach can be routinely applied, the predictive performance of specific drug modules must be evaluated in the target patient population to ensure reliability.
This lecture will explore strategies to assess the clinical viability of MIPD, aiming to equip healthcare professionals with practical guidance for its adoption and implementation.
Despite its potential, widespread adoption of MIPD in clinical settings remains limited. Before this approach can be routinely applied, the predictive performance of specific drug modules must be evaluated in the target patient population to ensure reliability.
This lecture will explore strategies to assess the clinical viability of MIPD, aiming to equip healthcare professionals with practical guidance for its adoption and implementation.
Biography
Dr. Nynke Jager is a hospital pharmacist and clinical pharmacologist at Radboud University Medical Center (Radboudumc) in Nijmegen, the Netherlands. She leads the laboratory and therapeutic drug monitoring (TDM) processes and serves as president of the hospital’s Antibiotics Committee.
Her research is centered on optimizing antimicrobial therapies, with a particular focus on critically ill patients. Dr. Jager is the principal investigator of several multicenter clinical trials, including the SNAP trial on the treatment of Staphylococcus aureus bacteremia and the HIT HARD trial, which explores antibiotic dosing strategies in ICU settings.
In addition, her work encompasses bioanalytical assay development, TDM implementation and model-informed precision dosing (MIPD), aiming to personalize and improve antibiotic therapy for better patient outcomes.
Dr Guangda Ma
University of Auckland
Implementing model-based dosing to improve clinical outcomes: Results of external evaluation of vancomycin popPK models and implementation in a NZ hospital
Abstract
Following model selection (1), we conducted a prospective before-after study to evaluate the effectiveness, safety, and acceptability of vancomycin management using an area under the curve (AUC)-target concentration dosingapproach, compared to historical trough therapeutic window dosing approach. AUCdosing used the web-based Bayesian dosing tool NextDose. Each cohort consisted of 200 patients from Auckland City and Starship Children’s hospitals.
The primary effectiveness endpoint compared the proportion of AUCss24 between 400-600 mg/L∙h in the AUC and trough guided cohorts. The secondary endpoint for effectiveness was based on reasons for vancomycin cessation. The primary safety endpoint compared the hazard of vancomycin-associated nephrotoxicity (VAN). and the secondary endpoint for safety was all-cause mortality.
Acceptable AUCss24 values were more likely to be achieved in the AUC guided cohort (59% vs 39.4%, P < 0.001). The hazard of VAN was lower in the AUC guided cohort (HR: 0.318; 95% CI: 0.123 – 0.823; P = 0.01). No difference was observed in clinical effectiveness based on reasons for vancomycin cessation nor in safety based on all-cause mortality. Prescribers accepted 87% of NextDose recommendations. A dose adjustment followed 34.9% of acceptable NextDose recommendations.
AUC guided vancomycin management, supported by NextDose, was associated with improved target attainment and marked reduction in VAN. This confirms the clinical benefits of a Bayesian model-based, target concentration intervention approach to dose individualisation.
The primary effectiveness endpoint compared the proportion of AUCss24 between 400-600 mg/L∙h in the AUC and trough guided cohorts. The secondary endpoint for effectiveness was based on reasons for vancomycin cessation. The primary safety endpoint compared the hazard of vancomycin-associated nephrotoxicity (VAN). and the secondary endpoint for safety was all-cause mortality.
Acceptable AUCss24 values were more likely to be achieved in the AUC guided cohort (59% vs 39.4%, P < 0.001). The hazard of VAN was lower in the AUC guided cohort (HR: 0.318; 95% CI: 0.123 – 0.823; P = 0.01). No difference was observed in clinical effectiveness based on reasons for vancomycin cessation nor in safety based on all-cause mortality. Prescribers accepted 87% of NextDose recommendations. A dose adjustment followed 34.9% of acceptable NextDose recommendations.
AUC guided vancomycin management, supported by NextDose, was associated with improved target attainment and marked reduction in VAN. This confirms the clinical benefits of a Bayesian model-based, target concentration intervention approach to dose individualisation.
Biography
I studied pharmacy and clinical pharmacometrics at the University of Auckland. My current research interests focus on the implementation and evaluation population models to improve clinical outcomes, clinical trial simulation, and model informed drug development.
Session chair
Iris Minichmayr
Medical University Of Vienna
James Morse
University of Auckland
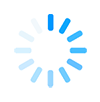